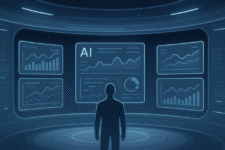
The artificial intelligence observability market is experiencing explosive growth, projected to reach $10.7 billion by 2033 with a compound annual growth rate of 22.5%. As AI adoption accelerates—with 78% of organizations now using AI in at least one business function, up from 55% just two years ago—effective monitoring has become mission-critical for ensuring reliability, transparency, and compliance.
Organizations deploying AI at scale face unique challenges including data drift, concept drift, and emergent behaviors that traditional monitoring tools weren’t designed to handle. Modern AI observability platforms combine the ability to track model performance with specialized features like bias detection, explainability metrics, and continuous validation against ground truth data.
This comprehensive guide explores the most powerful AI observability platforms available today, providing detailed information on capabilities, pricing, pros and cons, and recent developments to help you make an informed decision for your organization’s specific needs.
Comparison Table of Best AI Observability Tools
AI Tool | Best For | Price | Features |
---|---|---|---|
Arize AI | Comprehensive AI lifecycle monitoring | $50/mo | End-to-end AI visibility, OpenTelemetry support, LLM tracing |
Fiddler AI | Explainability & LLM security | Custom pricing | AI explainability, Trust Service, SOC 2/HIPAA compliant |
Superwise | ML model drift detection | Free + Usage-based | 100+ metrics, Alert correlation, Industry solutions |
Datadog | Infrastructure + AI unified view | $15/host/mo | Full-stack visibility, LLM tracing, Prompt clustering |
Dynatrace | Enterprise automation | $69/mo | Davis AI engine, Automated RCA, Topology mapping |
New Relic | Business-focused insights | $49/user | AI-driven insights, Business observability, 50+ capabilities |
WhyLabs | Privacy & open-source needs | Free | Privacy-first architecture, Real-time guardrails |
Grafana | Visualization & dashboards | $49/mo | GPU monitoring, Custom dashboards, Flexible deployment |
IBM Instana | Complex enterprise environments | $200/mo | Automated discovery, GenAI Runtime sensor, 1-second granularity |
Middleware | Cost-effective full-stack | Free + Pay-as-you-go | Unified timeline, GPT-4 integration, 60-75% cost savings |
*Pricing is in $USD
1. Arize AI
Founded in 2020, Arize AI has secured $131 million in funding, including a recent $70 million Series C round in February 2025. The company serves high-profile clients like Uber, DoorDash, and the U.S. Navy. Their platform provides end-to-end AI visibility with OpenTelemetry instrumentation, offering continuous evaluation capabilities with LLM-as-a-Judge functionality.
Arize’s strength lies in its purpose-built design specifically for AI rather than being adapted from traditional monitoring tools. The platform includes Arize AI Copilot for troubleshooting assistance and supports a comprehensive range of AI applications from traditional ML to LLMs and AI agents. Their approach to performance tracing allows teams to pinpoint model failures quickly, while their strong partner ecosystem integrates seamlessly with major cloud platforms.
Pros and Cons
- Comprehensive coverage across the AI application lifecycle
- Built on open standards with open-source options
- Purpose-built for AI rather than adapted from traditional tools
- Strong partner ecosystem with major cloud platforms
- Enterprise pricing might be expensive for smaller organizations
- Learning curve for those new to MLOps
- Limited API documentation for some use cases
Pricing (USD)
• AX Pro: $50/month for 3 users, 2 models/apps, 10,000 spans
• AX Enterprise: Custom pricing for advanced needs
• Free: Open-source Phoenix option available
2. Fiddler AI
Fiddler AI has raised $68.6 million, including an $18.6 million Series B Prime round in December 2024. The company positions itself as a pioneer in AI Observability and AI Safety. Their platform provides real-time monitoring of model performance and data drift along with industry-leading explainability tools for model predictions and behaviors.
Fiddler’s standout feature is their comprehensive fairness and bias assessment frameworks alongside their Fiddler Trust Service for scoring and monitoring LLM applications. The platform offers cutting-edge LLM observability capabilities with Fiddler Guardrails for prompt/response moderation. With enterprise-grade security including SOC 2 Type 2 and HIPAA compliance, Fiddler has established itself as a trusted solution for organizations with stringent compliance requirements.
Pros and Cons
- Industry-leading explainability capabilities
- Enterprise-grade security and compliance
- LLM observability with Trust Service
- Extensive integration capabilities
- Steep learning curve for those new to AI monitoring
- Complex pricing structure
- Significant computational resource requirements
Pricing (USD)
- Lite Plan: For individual practitioners
- Standard/Business Plan: For teams with business KPI alignment
- Premium/Enterprise Plan: For complex enterprise needs
3. Superwise
Superwise excels at data quality monitoring and pipeline validation with comprehensive drift detection across various data types. The platform has gained recognition for their intelligent incident correlation, which significantly reduces alert fatigue. The platform’s bias and fairness monitoring capabilities ensure compliance with regulatory requirements while providing segment-level performance insights. Their industry-specific AI solutions demonstrate a deep understanding of sector-specific challenges.
Pros and Cons
- Comprehensive monitoring with 100+ pre-built metrics
- Intelligent incident correlation to reduce alert fatigue
- Platform-agnostic and model-agnostic design
- Complex implementation for less mature organizations
- Enterprise focus may not suit smaller teams
- Limited public case studies
- Recent organizational changes creating some uncertainty
Pricing (USD)
- Community Edition: Free for up to 3 models and 3 users
- Scale and Enterprise Plans: Usage-based pricing
- Volume discounts automatically applied as usage grows
4. Datadog
Datadog is a leading cloud monitoring platform that has evolved its comprehensive AI observability solution to help teams monitor, improve, and secure LLM applications. Their integrated approach combines AI monitoring with existing infrastructure monitoring for a unified view of system performance. The platform provides end-to-end tracing of LLM chains with sophisticated prompt and response clustering capabilities.
One of Datadog’s standout features is its seamless integration with existing infrastructure monitoring, allowing teams to correlate AI performance with underlying system metrics. The platform includes advanced clustering for prompt/response analysis and built-in security features through integration with Sensitive Data Scanner. Their comprehensive visibility approach ensures teams can monitor everything from application performance to AI model behavior in a single dashboard.
Pros and Cons
- Comprehensive visibility across the entire stack
- Seamless integration with existing infrastructure
- Advanced clustering for prompt/response analysis
- Built-in security features
- Potentially expensive for high data volumes
- More complex setup than specialized tools
- Requires existing Datadog infrastructure for full value
- Potentially overwhelming UI for new users
Pricing (USD)
- Free Tier: Limited features, 5 hosts, 100GB log management
- Pro Plan: $15/host/month
- Enterprise Plan: $23/host/month
5. Dynatrace
Dynatrace offers a unified observability and security platform powered by their Davis AI engine, which combines predictive, causal, and generative AI capabilities for precise answers and intelligent automation. Their hypermodal AI approach sets them apart by integrating multiple AI methodologies into a cohesive platform that can predict, explain, and generate insights simultaneously.
The platform’s strength lies in its automated root cause analysis with natural language explanations through Davis CoPilot. Davis AI provides real-time problem detection and resolution while creating end-to-end topology maps that help teams visualize complex system dependencies. The platform’s sophisticated forecasting capabilities help organizations plan for capacity and prevent issues before they impact users.
Pros and Cons
- Mature AI engine with years of development
- Precise root cause analysis capabilities
- Integrated security and observability
- Strong predictive capabilities
- Higher learning curve than some competitors
- Premium pricing may exclude smaller organizations
- Complex deployment for large implementations
- Resource-intensive platform
Pricing (USD)
- Full-Stack Monitoring: ~$69/month/host (annual)
- Infrastructure Monitoring: ~$21/month/host (annual)
- Digital Experience Monitoring: Per user/visit pricing
6. New Relic
New Relic has transformed from a traditional reliability platform into a comprehensive Intelligent Observability Platform that drives business growth and developer velocity. Their AI Engine combines compound and agentic AI technologies to deliver contextual insights across the entire stack. The platform stands out for connecting technical metrics with business outcomes through features like Pathpoint Plus for business observability.
What distinguishes New Relic is their focus on business value rather than just technical metrics. Their Response Intelligence feature contextualizes metrics to help teams understand the business impact of technical issues. With 50+ platform capabilities and extensive integrations with developer tools, New Relic provides a full-stack solution that scales from small teams to enterprise organizations.
Pros and Cons
- Full-stack observability with 50+ platform capabilities
- AI-driven insights with predictive capabilities
- Business-focused observability
- Extensive integrations with developer tools
- Feature richness creating complexity for new users
- Performance issues with large data volumes
- Limited customization in some areas
- Higher learning curve for advanced features
Pricing (USD)
- Free Tier: 100GB data, 1 full platform user
- Standard Tier: $49/core user, 100GB data
- Pro Tier: $349/full platform user
- Enterprise Tier: Custom pricing
7. WhyLabs
WhyLabs provides AI observability and security tools that became open-source under the Apache 2 license in January 2025, allowing organizations to run the platform on their own infrastructure. Their approach emphasizes privacy-first architecture with real-time guardrails for GenAI applications. The platform includes built-in prompt injection and jailbreak detection with customizable threat detection rules.
The open-source nature of WhyLabs gives organizations complete control over their monitoring infrastructure while maintaining privacy compliance. Their platform offers comprehensive security features with low-latency threat detection under 300ms. However, organizations should note that while the platform is free, it requires a Highcharts license for visualization.
Pros and Cons
- Available as open-source software
- Privacy-first approach with no data leaving environment
- Comprehensive security features
- Low-latency threat detection (under 300ms)
- Requires Highcharts license for visualization
- Complex setup for some deployment scenarios
- Younger platform than enterprise alternatives
Pricing (USD)
- Open Source: Free under Apache 2 license
- Previous SaaS tiers being phased out
8. Grafana
Grafana Labs offers an open-source platform for visualizing and analyzing data, with AI Observability capabilities specifically designed for monitoring generative AI applications, LLMs, vector databases, and GPUs. Their platform integrates with OpenLIT SDK to provide comprehensive monitoring dashboards that excel at visualizing complex AI metrics. Grafana’s strength lies in its exceptional visualization capabilities and flexible deployment options.
The platform’s visualization-first approach makes it easier for teams to understand AI system performance at a glance. With support for GPU performance monitoring, token usage tracking, and cost monitoring, Grafana provides a comprehensive view of AI application health. Their modular architecture allows teams to customize dashboards and create tailored monitoring solutions for specific AI workloads.
Pros and Cons
- Built on open-source technologies
- Easy integration with existing deployments
- Excellent visualization capabilities
- Flexible deployment options
- Requires technical expertise for effective setup
- Less automated remediation than alternatives
- May require custom development for advanced cases
- Dashboard creation learning curve
Pricing (USD)
- Free: 10k metrics, 50GB logs, 50GB traces
- Pro: $49/month with 100GB logs/traces, 20k metrics
- Advanced/Enterprise: Custom pricing (starts at $299/month)
9. IBM Instana
IBM Instana provides automated real-time observability for complex cloud environments, with AI-driven capabilities to help teams troubleshoot issues and optimize application performance. Their platform offers automated discovery across hybrid environments combined with real-time monitoring at 1-second granularity.
The platform excels in complex enterprise environments where automated discovery and fast time-to-value are crucial. Their GenAI Runtime sensor enables comprehensive monitoring of AI workloads while maintaining IBM’s high standards for security and compliance.
Pros and Cons
- Comprehensive visibility across complex environments
- Excellent automated discovery
- Fast time-to-value with minimal configuration
- Strong IBM ecosystem support
- Premium pricing may exclude smaller organizations
- Steeper learning curve for advanced features
- Strongest within IBM ecosystem
Pricing (USD)
- Observability Essentials: ~$20/MVS/month
- Observability Standard: ~$75/MVS/month
- Enterprise: Custom
10. Middleware
Middleware provides a full-stack cloud observability platform that unifies metrics, logs, traces, and events into a single timeline, using AI for anomaly detection and error resolution. Their innovative unified timeline approach helps teams understand the sequence of events leading to issues more intuitively.
Middleware’s cost-effective approach makes it attractive for organizations looking to optimize their observability budget without sacrificing functionality. Their single-command installation simplifies deployment while their AI-powered analysis provides advanced insights comparable to enterprise platforms.
Pros and Cons
- Unified timeline view of all observability data
- Simple installation and setup
- Cost-effective compared to enterprise alternatives
- Advanced AI-powered analysis
- Newer platform with less market presence
- Less extensive documentation
- Smaller community for knowledge sharing
- Limited third-party integrations
Pricing (USD)
- Free Forever Plan: Limited but functional
- Pay As You Go: Usage-based pricing
- Enterprise: Custom pricing
How to Choose the Right AI Observability Tool
Selecting the appropriate AI observability solution requires evaluating several critical factors:
1. Assess your organization’s AI maturity
Before evaluating tools, understand your organization’s current AI deployments, critical risks, regulatory requirements, and technical capabilities. Organizations with multiple production models have different needs than those just starting their AI journey.
2. Define clear requirements
Identify the specific metrics you need to track, establish performance baselines, determine alert priorities, and clarify reporting needs for stakeholders. Consider what types of models you’re monitoring (traditional ML, LLMs, computer vision) and their specific observability requirements.
3. Evaluate technical compatibility
Review your existing technology stack and identify integration points. 97% of IT decision-makers actively manage observability costs, making it crucial to select tools that integrate well with your infrastructure while optimizing expenses.
As AI adoption accelerates across industries, the need for robust observability becomes increasingly critical. The tools highlighted in this guide represent the cutting edge of AI monitoring technology, each offering unique approaches to ensuring reliability, performance, and compliance.
FAQ (AI Observability Tools)
1. What are the key features to look for in an AI observability tool?
Essential features include real-time model performance monitoring, drift detection, explainability capabilities, automated anomaly detection, integration with existing infrastructure, and guardrails for AI safety.
2. How does anomaly detection work in AI observability tools?
AI observability tools use statistical models and machine learning algorithms to establish baseline behaviors for AI systems. When metrics deviate significantly from normal patterns, the tools trigger alerts and provide context for investigation.
3. Which AI observability tool offers the best integration with existing systems?
Datadog leads in integration capabilities through its extensive ecosystem and ability to seamlessly connect AI monitoring with existing infrastructure observability. Its unified platform approach eliminates data silos.
4. How do AI observability tools help in reducing model bias?
These tools continuously monitor fairness metrics, demographic parity, and equal opportunity indicators. They flag potential bias in real-time and provide visualization tools to identify which data segments experience disparate outcomes.
5. What are the benefits of using AI-powered observability tools like Arize AI?
Arize AI offers purpose-built AI monitoring with comprehensive lifecycle coverage, open-source flexibility, and automated troubleshooting. Its advanced tracing capabilities and cloud platform integrations accelerate debugging for development teams.
The post 10 Best AI Observability Tools (May 2025) appeared first on Unite.AI.