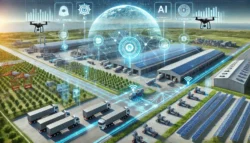
Before we explore the sustainability aspect, let’s briefly recap how AI is already revolutionizing global logistics:
Route Optimization
AI algorithms are transforming route planning, going far beyond simple GPS navigation. For instance, UPS’s ORION (On-Road Integrated Optimization and Navigation) system uses advanced algorithms to optimize delivery routes. It considers factors like traffic patterns, package priorities, and promised delivery windows to create the most efficient routes. The result? UPS saves about 10 million gallons of fuel annually, reducing both costs and emissions.
As a product manager at Amazon, I worked on similar systems that not only optimized last-mile delivery but also coordinated with warehouse operations to ensure the right packages were loaded in the optimal order. This level of integration between different parts of the supply chain is only possible with AI’s ability to process vast amounts of data in real-time.
Supply Chain Visibility
AI-powered tracking systems are providing unprecedented visibility into the supply chain. During my time at Maersk, we developed a system that used IoT sensors and AI to provide real-time tracking of containers. This wasn’t just about location – the system monitored temperature, humidity, and even detected unauthorized access attempts.
For example, when shipping sensitive pharmaceuticals, any temperature deviation could be immediately detected and corrected. The AI didn’t just report issues; it predicted potential problems based on weather forecasts and historical data, allowing for proactive interventions. This level of visibility and predictive capability significantly reduced losses and improved customer satisfaction.
Predictive Maintenance
AI is revolutionizing how we approach equipment maintenance in logistics. At Amazon, we implemented machine learning models that analyzed data from sensors on conveyor belts, sorting machines, and delivery vehicles. These models could predict when a piece of equipment was likely to fail, allowing for maintenance to be scheduled during off-peak hours.
For instance, our system once predicted a potential failure in a crucial sorting machine 48 hours before it would have occurred. This early warning allowed us to perform maintenance without disrupting operations, potentially saving millions in lost productivity and late deliveries.
Demand Forecasting
AI is revolutionizing how we predict demand in the logistics industry. During my time at Amazon, we developed machine learning models that analyzed not just historical sales data, but also factors like social media trends, weather forecasts, and even upcoming events in different regions.
For instance, our system once predicted a spike in demand for certain electronics in a specific region, correlating it with a local tech convention that wasn’t on our radar. This allowed us to adjust inventory and staffing levels accordingly, avoiding stockouts and ensuring smooth operations during the event.
Last-Mile Delivery Optimization
The final leg of delivery, known as last-mile, is often the most challenging and costly part of the logistics process. AI is making significant inroads here too. At Amazon, we worked on AI systems that optimized not just routes, but also delivery methods.
For example, in urban areas, the system would analyze traffic patterns, parking availability, and even building access methods to determine whether a traditional van delivery, a bicycle courier, or even a drone delivery would be most efficient for each package. This granular level of optimization resulted in faster deliveries, lower costs, and reduced urban congestion.
The Product Manager’s Dilemma
As product managers in the logistics industry, we’re tasked with driving innovation and efficiency. AI offers unprecedented opportunities to do just that. However, we now face a critical dilemma:
Efficiency Gains
On one hand, AI-powered supply chains are more optimized than ever before. They reduce waste, minimize fuel consumption, and potentially lower the overall carbon footprint of logistics operations. The route optimization algorithms we implement can significantly reduce unnecessary mileage and emissions.
Environmental Costs
On the other hand, we can’t ignore the environmental cost of AI itself. The training and operation of large AI models consume enormous amounts of energy, contributing to increased power demands and, by extension, carbon emissions.
This raises a pivotal question for us as product managers: How do we balance the sustainability gains from AI-optimized supply chains against the environmental impact of the AI systems themselves?
New Responsibilities for Product Managers
In the age of AI, our role as product managers has expanded. We now have the added responsibility of considering sustainability in our decision-making processes. This involves:
- Life Cycle Analysis: We must consider the entire lifecycle of our AI-powered products, from development to deployment and maintenance, assessing their environmental impact at each stage.
- Efficiency Metrics: Alongside traditional KPIs, we need to incorporate sustainability metrics into our product evaluations. This might include energy consumption per optimization, carbon footprint reduction, or sustainability ROI.
- Vendor Selection: When choosing AI solutions or cloud providers, energy efficiency and use of renewable energy sources should be key selection criteria.
- Innovation Focus: We should prioritize and allocate resources to projects that not only improve operational efficiency but also enhance sustainability.
- Stakeholder Education: We need to educate our teams, executives, and clients about the importance of sustainable AI practices in logistics.
Industry Leaders Paving the Way
As product managers, we can learn a lot from how industry giants are tackling the challenge of balancing AI efficiency with sustainability. Let me share some insights from my experiences at Amazon and Maersk.
Amazon Web Services (AWS): Pioneering Sustainable Cloud Computing
During my time at Amazon, I witnessed firsthand the company’s commitment to reducing the power consumption of its AWS infrastructure, which hosts numerous AI and machine learning workloads for logistics and other industries. AWS has been implementing several strategies to improve energy efficiency:
- Renewable Energy: AWS has committed to powering its operations with 100% renewable energy by 2025. As of 2023, they’ve already reached 85% renewable energy use.
- Custom Hardware: Amazon designs custom chips like the AWS Graviton processors, which are up to 60% more energy-efficient than comparable x86-based instances for the same performance.
- Water Conservation: AWS has implemented innovative cooling technologies and uses reclaimed water for cooling in many regions, significantly reducing water consumption.
- Machine Learning for Efficiency: Ironically, AWS uses AI itself to optimize the energy efficiency of its data centers, predicting and adjusting for computing loads to minimize energy waste.
As product managers in logistics, we can leverage these advancements by choosing energy-efficient cloud services and advocating for the use of sustainable computing resources in our AI implementations.
Maersk: Setting New Standards for Shipping Emissions
At Maersk, I am part of the team working towards ambitious environmental goals that are reshaping the shipping industry. Maersk has set industry-leading emission targets:
- Net Zero Emissions by 2040: Maersk aims to achieve net zero greenhouse gas emissions across its entire business by 2040, a decade ahead of the Paris Agreement goals.
- Near-Term Targets: By 2030, Maersk aims to reduce its CO2 emissions per transported container by 50% compared to 2020 levels.
- Green Corridor Initiatives: Maersk is establishing specific shipping routes as “green corridors,” where zero-emission solutions are supported and demonstrated.
- Investment in New Technologies: The company is investing in methanol-powered vessels and exploring other alternative fuels to reduce emissions.
As product managers in logistics, we played a crucial role in aligning our AI and technology initiatives with these sustainability goals. For instance:
- Route Optimization: We developed AI algorithms that not only optimized for speed and cost but also for fuel efficiency and emissions reduction on regular shipping routes.
- Predictive Maintenance: Our AI models for predictive maintenance helped ensure ships were operating at peak efficiency, further reducing fuel consumption and emissions.
- Supply Chain Visibility: We created tools that provided customers with detailed emissions data for their shipments, encouraging more sustainable choices.
The Path Forward
Despite the challenges, I believe that the implementation of AI in logistics remains a worthy undertaking. As product managers, we have a unique opportunity to drive positive change. Here’s why and how we can move forward:
Continuous Improvement
As product managers, we’re in a unique position to drive the evolution of more energy-efficient AI solutions. The same optimization principles we apply to supply chains can be directed towards improving the efficiency of our AI systems. This means constantly evaluating and refining our AI models, not just for performance but for energy efficiency. We should work closely with data scientists and engineers to develop models that achieve high accuracy with less computational power. This might involve techniques like model pruning, quantization, or using more efficient neural network architectures. By making energy efficiency a key performance indicator for our AI products, we can drive innovation in this crucial area.
Net Positive Impact
While AI systems do consume significant energy, the scale of optimization they bring to global logistics likely results in a net positive environmental impact. Our role is to ensure and maximize this positive balance. This requires a holistic view of our operations. We need to implement comprehensive monitoring systems that track both the energy consumption of our AI systems and the energy savings they generate across the supply chain. By quantifying this net impact, we can make data-driven decisions about which AI initiatives to prioritize. Moreover, we can use this data to create compelling narratives about the sustainability benefits of our products, which can be a powerful tool in stakeholder communications and marketing efforts.
Catalyst for Innovation
The sustainability challenge is driving innovation in green computing and renewable energy. As product managers, we can champion and guide this innovation within our organizations. This might involve partnering with green tech startups, allocating a budget for sustainability-focused R&D, or creating cross-functional “green teams” to tackle sustainability challenges. We should also stay abreast of emerging technologies like quantum computing or neuromorphic chips that promise vastly improved energy efficiency. By positioning ourselves at the forefront of these innovations, we can ensure our products are not just keeping pace with sustainability trends but setting new standards for the industry.
Long-term Vision
We need to take a long-term view, considering how our product decisions today will impact sustainability in the future. This includes anticipating the transition to cleaner energy sources, which will decrease the environmental cost of powering AI systems over time. As product managers, we should be advocating for and planning this transition within our own operations. This might involve setting ambitious timelines for shifting to renewable energy sources, or designing our systems to be adaptable to future energy technologies. We should also be thinking about the full lifecycle of our products, including how they can be sustainably decommissioned or upgraded at the end of their life. By embedding this long-term thinking into our product strategies, we can create truly sustainable solutions that stand the test of time.
Competitive Advantage
Sustainable AI practices can become a significant differentiator in the market. Product managers who successfully balance efficiency and sustainability will lead the industry forward. This is not just about doing good for the planet – it’s about positioning our products for future success. Customers, particularly in the B2B space, are increasingly prioritizing sustainability in their purchasing decisions. By making sustainability a core feature of our products, we can tap into this growing market demand. We should be working with our marketing teams to effectively communicate our sustainability efforts, potentially pursuing certifications or partnerships that validate our green credentials. Moreover, as regulations around AI and sustainability evolve, products with strong environmental performance will be better positioned to comply with future requirements.
Ethical Responsibility
As leaders in the field of AI and logistics, we have an ethical responsibility to consider the broader impacts of our work. This goes beyond just environmental concerns to include social and economic impacts as well. We should be thinking about how our AI systems affect jobs, privacy, and equity in the supply chain. By taking a proactive approach to these ethical considerations, we can build trust with our stakeholders and create products that contribute positively to society as a whole. This might involve implementing ethical AI frameworks, conducting regular impact assessments, or engaging with a diverse range of stakeholders to understand different perspectives on our work.
Collaboration and Knowledge Sharing
The challenges of sustainable AI in logistics are too big for any one company to solve alone. As product managers, we should be fostering collaboration and knowledge sharing within the industry. This could involve participating in industry consortiums, contributing to open-source projects, or sharing best practices at conferences and in publications. By working together, we can accelerate the development of sustainable AI solutions and create standards that lift the entire industry. Moreover, by positioning ourselves as thought leaders in this space, we can enhance our professional reputations and the reputations of our companies.
Conclusion
As product managers in the logistics industry, we have a unique opportunity – and responsibility – to shape the future of sustainable, AI-powered logistics. The challenge of balancing AI’s benefits with its energy consumption is driving innovation in green computing and renewable energy, with potential benefits far beyond our sector.
By thoughtfully considering both the efficiency gains and environmental costs of AI in our product decisions, we can drive innovation that not only optimizes operations but also contributes to a more sustainable future for global logistics. It’s a complex challenge, but one that offers immense potential for those willing to lead the way.
The future of logistics is not just about being faster and more efficient – it’s about being smarter and more sustainable. As product managers, it’s our job to make that future a reality.
The post AI, Sustainability, and Product Management in Global Logistics: Navigating the New Frontier appeared first on Unite.AI.