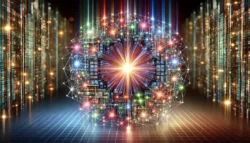
The human brain is the biggest energy consumer in the body, and we tend to reduce energy consumption and try to minimize cognitive load. We are inherently lazy, always seeking ways to automate even the most minor tasks. True automation means not having to lift a finger to get things done. This is where agentic AI shines, the term “agentic” is derived from the concept of an “agent,” which in AI parlance, is an entity capable of performing tasks independently. Unlike traditional AI systems that operate based on pre-defined rules and datasets, agentic AI possesses the capability to make autonomous decisions, adapt to new environments, and learn from its interactions. We will explore the intricacies of agentic AI, exploring its potential and challenges.
Understanding the Key Components of Agentic AI
Agentic AI systems designed to act autonomously, making decisions without human intervention. These systems are characterized by their ability to perceive their environment, reason for it, and take actions to achieve specific goals.
- Perception: Agentic AI systems are equipped with advanced sensors and algorithms that allow them to perceive their surroundings. This includes visual, auditory, and tactile sensors that provide a comprehensive understanding of the environment.
- Reasoning: At the core of agentic AI is its reasoning capability. These systems use sophisticated algorithms, including machine learning and deep learning, to analyze data, identify patterns, and make informed decisions. This reasoning process is dynamic, allowing the AI to adapt to new information and changing circumstances.
- Communication: An AI co-worker is a collection of agents under a supervisor, executing specific functions end to end. These agents coordinate with each other and bring humans into the loop in case of escalations or pre-defined verification to complete a given process.
- Reactive and Proactive Approach: Agentic AI systems can respond to immediate stimuli (reactive) and anticipate future needs or changes (proactive). This dual capability ensures they can handle both current and future challenges effectively.
- Action: Once a decision is made, agentic AI systems can execute actions autonomously. This could range from physical actions, such as navigating a robot through a complex environment, to digital actions, like managing a financial portfolio.
How Agentic AI Might Work in Real Life
To illustrate how agentic AI might function in real-world scenarios, consider the following example involving three separate AI co-workers performing tasks in tandem to accomplish automatic, streamlined data aggregation:
- AI Marketing Analyst: This AI system gathers and analyzes data from various sources, including website interactions and social media. It identifies patterns and insights that can be used to understand customer behavior and market trends.
- AI Business Development Executive: Utilizing the intelligence provided by the AI Marketing Analyst, this AI system engages with leads more effectively. For instance, when a visitor comes to a website, the AI Business Development Executive can identify the visitor’s buying intent based on the data from the AI Analyst. This allows for more focused and personalized engagements, increasing the likelihood of converting leads into customers.
- AI Customer Care Executive: The data from social media listening and other sources analyzed by the AI Marketing Analyst is also used by the AI Customer Care Executive. This AI system identifies common problems and concerns that customers are facing, often from a competitive perspective. Armed with this information, the sales team can utilize these insights to address customer issues proactively and explore upselling opportunities.
Challenges and Ethical Considerations
While the potential of agentic AI is immense, it also presents several challenges and ethical considerations:
- Safety and Reliability: Ensuring that agentic AI systems operate safely and reliably is paramount. These systems must be rigorously tested to prevent malfunctions that could lead to accidents or unintended consequences.
- Transparency: The decision-making processes of agentic AI systems can be complex and opaque. It is crucial to develop methods for making these processes transparent and understandable to humans, especially in critical applications like healthcare and finance.
- Ethical Decision-Making: Agentic AI systems must be programmed with ethical guidelines to ensure they make decisions that align with societal values. This includes addressing issues like bias, fairness, and accountability.
- Regulation and Governance: As agentic AI becomes more prevalent, there will be a need for robust regulatory frameworks to govern its use. This includes establishing standards for safety, privacy, and ethical behavior.
Comparing Agentic AI to Traditional RPA
Traditional Robotic Process Automation (RPA) platforms were primarily focused on building bots that interacted predominantly through user interfaces (UI). Their strength lies in automating repetitive tasks by simulating human interaction with UIs; however, as we move toward an agentic approach paradigm shifts significantly.
In an agentic framework focus broadens beyond UI interactions encompassing back-end automation decision-making instead relying solely on UI automation emphasis shifts toward leveraging APIs integrating technologies like Large Language Models (LLMs) enabling productive intelligent decision-driven workflows.
Key differentiators include:
- Enhanced Capability Set: Agentic introduces higher level capability extending beyond traditional RPA functionalities including advanced Intelligent Document Processing (IDP) integration LLMs ability manage complex workflows decision-making capabilities driven by.
- Technology Convergence: AI co-workers embrace the strategy creating ecosystem where various technologies seamlessly interact unlike earlier RPA systems primarily depended on UI-based interactions model allows direct integration coordination among components APIs other systems.
- End-to-End Automation Without Human Supervision: An AI co-worker, comprising a collection of agents under a supervisor, manages entire workflows autonomously. These agents coordinate with each other and involve humans only for escalations or pre-defined verification, ensuring true end-to-end automation.
The Future of Agentic AI
The agentic approach is not entirely new. In fact, it has been a core part of AI development for several years. The concept involves creating AI co-workers, each functioning as a specific agent—or more accurately, a collection of agents. An AI co-worker is essentially a team of agents working together under a unified framework designed to coordinate seamlessly with other similar teams. For instance, one AI co-worker might specialize in Intelligent Document Processing (IDP) with its own agents handling specific subtasks. These teams, each with their specialized agents and supervisors, can work together to achieve broader goals.
In conclusion, agentic AI represents a significant leap forward in artificial intelligence, offering unprecedented opportunities for innovation and efficiency while demanding careful navigation to ensure its benefits are realized in a safe, transparent, and ethical manner.
The post Agentic AI: The Future of Autonomous Decision-Making appeared first on Unite.AI.