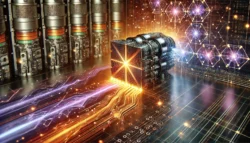
The rapid rise of Artificial Intelligence (AI) has transformed numerous sectors, from healthcare and finance to energy management and beyond. However, this growth in AI adoption has resulted in a significant issue of energy consumption. Modern AI models, particularly those based on deep learning and neural networks, are incredibly power-hungry. Training a single large-scale model can use as much energy as multiple households consume yearly, leading to significant environmental impact. As AI becomes more embedded in our daily lives, finding ways to reduce its energy usage is not just a technical challenge; it’s an environmental priority.
The Tsetlin Machine offers a promising solution. Unlike traditional neural networks, which rely on complex mathematical computations and massive datasets, Tsetlin Machines employ a more straightforward, rule-based approach. This unique methodology makes them easier to interpret and significantly reduces energy consumption.
Understanding the Tsetlin Machine
The Tsetlin Machine is an AI model that reimagines learning and decision-making. Unlike neural networks, which rely on layers of neurons and complex computations, Tsetlin Machines use a rule-based approach driven by simple Boolean logic. We can think of Tsetlin Machines as machines that learn by creating rules to represent data patterns. They operate using binary operations, conjunctions, disjunctions, and negations, making them inherently simpler and less computationally intensive than traditional models.
TMs operate on the principle of reinforcement learning, using Tsetlin Automata to adjust their internal states based on feedback from the environment. These automata function as state machines that learn to make decisions by flipping bits. As the machine processes more data, it refines its decision-making rules to improve accuracy.
One main feature that differentiates Tsetlin Machines from neural networks is that they are easier to understand. Neural networks often work like “black boxes,” giving results without explaining how they got there. In contrast, Tsetlin Machines create clear, human-readable rules as they learn. This transparency makes Tsetlin Machines easier to use and simplifies the process of fixing and improving them.
Recent advancements have made Tsetlin Machines even more efficient. One essential improvement is deterministic state jumps, which means the machine no longer relies on random number generation to make decisions. In the past, Tsetlin Machines used random changes to adjust their internal states, which was only sometimes efficient. By switching to a more predictable, step-by-step approach, Tsetlin Machines now learn faster, respond more quickly, and use less energy.
The Current Energy Challenge in AI
The rapid growth of AI has led to a massive increase in energy use. The main reason is the training and deployment of deep learning models. These models, which power systems like image recognition, language processing, and recommendation systems, need vast amounts of data and complex math operations. For example, training a language model like GPT-4 involves processing billions of parameters and can take days or weeks on powerful, energy-hungry hardware like GPUs.
A study from the University of Massachusetts Amherst shows the significant impact of AI’s high energy consumption. Researchers found that training a single AI model can emit over 626,000 pounds of CO₂, about the same as the emissions from five cars over their lifetimes. This large carbon footprint is due to the extensive computational power needed, often using GPUs for days or weeks. Furthermore, the data centers hosting these AI models consume a lot of electricity, usually sourced from non-renewable energy. As AI use becomes more widespread, the environmental cost of running these power-hungry models is becoming a significant concern. This situation emphasizes the need for more energy-efficient AI models, like the Tsetlin Machine, which aims to balance strong performance with sustainability.
There is also the financial side to consider. High energy use means higher costs, making AI solutions less affordable, especially for smaller businesses. This situation shows why we urgently need more energy-efficient AI models that deliver strong performance without harming the environment. This is where the Tsetlin Machine comes in as a promising alternative.
The Tsetlin Machine’s Energy Efficiency and Comparative Analysis
The most notable advantage of Tsetlin Machines is their energy efficiency. Traditional AI models, especially deep learning architectures, require extensive matrix computations and floating-point operations. These processes are computationally intensive and result in high energy consumption. In contrast, Tsetlin Machines use lightweight binary operations, significantly reducing their computational burden.
To quantify this difference, let us consider the work by Literal Labs, a company leader of Tsetlin Machines applications. Literal Labs found that Tsetlin Machines can be up to 10,000 times more energy-efficient than neural networks. In tasks like image recognition or text classification, Tsetlin Machines can match the accuracy of traditional models while consuming only a fraction of the power. This makes them especially useful for energy-constrained environments, such as IoT devices, where saving every watt of power is critical.
Moreover, Tsetlin Machines are designed to operate efficiently on standard, low-power hardware. Unlike neural networks that often require specialized hardware like GPUs or TPUs for optimal performance, Tsetlin Machines can function effectively on CPUs. This reduces the need for expensive infrastructure and minimizes the overall energy footprint of AI operations. Recent benchmarks support this advantage, demonstrating that Tsetlin Machines can handle various tasks from anomaly detection to language processing using far less computational power than their neural network counterparts.
Comparing Tsetlin Machines with neural networks shows a clear difference in energy use. Neural networks require significant energy during both training and inference. They often need specialized hardware, which increases both environmental and financial costs. Tsetlin Machines, however, use simple rule-based learning and binary logic, resulting in much lower computational demands. This simplicity enables Tsetlin Machines to scale well in energy-limited settings like edge computing or IoT.
While neural networks may outperform Tsetlin Machines in some complex tasks, Tsetlin Machines excel where energy efficiency and interpretability matter most. However, they do have limitations. For example, Tsetlin Machines may struggle with extremely large datasets or complex problems. To address this, ongoing research is exploring hybrid models that combine the strengths of Tsetlin Machines with other AI techniques. This approach could help overcome current challenges and broaden their use cases.
Applications in the Energy Sector
Tsetlin Machines have substantially impacted the energy sector, where efficiency is of utmost significance. Below are some critical applications:
Smart Grids and Energy Management
Modern smart grids use real-time data to optimize energy distribution and predict demand. Tsetlin Machines analyzes consumption patterns, detects anomalies, and forecasts future energy needs. For example, in the UK’s National Grid, Tsetlin Machines assists in predictive maintenance by identifying potential failures before they happen, preventing costly outages and reducing energy waste.
Predictive Maintenance
In industries where machinery is vital, unexpected failures can waste energy and cause downtime. Tsetlin Machines analyzes sensor data to predict when maintenance is needed. This proactive approach ensures that machines run efficiently, reducing unnecessary power consumption and extending the lifespan of equipment.
Renewable Energy Management
Managing renewable energy sources like solar and wind power requires balancing production with storage and distribution. Tsetlin Machines forecasts energy generation based on weather patterns and optimizes storage systems to meet demand efficiently. Accurate predictions from Tsetlin Machines help create a more stable and sustainable energy grid, reducing reliance on fossil fuels.
Recent Developments and Innovations
The domain of Tsetlin Machine research is dynamic, with continuous innovations to improve performance and efficiency. Recent developments include the creation of multi-step finite-state automata, allowing Tsetlin Machines to handle more complex tasks with improved accuracy. This advancement expands the range of problems Tsetlin Machines can tackle, making them applicable to scenarios previously dominated by neural networks.
Additionally, researchers have introduced methods to reduce reliance on random number generation within Tsetlin Machines, opting for deterministic state changes instead. This shift speeds up the learning process, decreases computational requirements, and, most importantly, reduces energy consumption. As researchers refine these mechanisms, Tsetlin Machines are becoming increasingly competitive with more traditional AI models, particularly in domains where low power consumption is a priority.
The Bottom Line
The Tsetlin Machine is more than just a new AI model. It represents a shift toward sustainability in technology. Its focus on simplicity and energy efficiency challenges the idea that powerful AI must come with a high environmental cost.
Alongside the continuous AI developments, Tsetlin Machines offer a path forward where advanced technology and environmental responsibility go hand in hand. This approach is a technical breakthrough and a step toward a future where AI serves humanity and the planet. In conclusion, embracing Tsetlin Machines could be essential to building a more innovative, greener world.
The post A Game-Changer for AI: The Tsetlin Machine’s Role in Reducing Energy Consumption appeared first on Unite.AI.