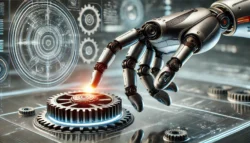
The World Quality Report 2024-25 by OpenText sheds light on groundbreaking trends shaping Quality Engineering (QE) and testing practices globally. With over 1,775 executives surveyed across 33 countries, the report uncovers how AI, automation, and sustainability are transforming the landscape of quality assurance. As AI technology progresses, organizations are being called to adopt new, innovative solutions for QE, especially as Generative AI (Gen AI) takes center stage.
We will explore the report’s findings, emphasizing key trends in QE, automation, and AI, and providing actionable insights for organizations ready to embrace the future of quality engineering.
The Rise of AI in Quality Engineering
One of the report’s least striking revelations is the rapid adoption of AI in QE. A staggering 71% of organizations have integrated AI and Gen AI into their operations, up from 34% in previous years. This shift marks a pivotal moment in the industry, with AI set to revolutionize various aspects of QE, from test automation to data quality management.
AI’s impact is particularly profound in test automation, where 73% of respondents cite AI and machine learning (ML) as key drivers of progress. Cloud-native technologies and robotic process automation (RPA) follow closely behind, with 67% and 66%, respectively, leveraging these advancements. The speed and efficiency of automation are improving dramatically, allowing organizations to reduce manual efforts and increase testing scope.
For instance, 72% of organizations report that Gen AI has accelerated their test automation processes, while 68% highlight easier integrations, enabling a seamless fit into existing development pipelines. By automating repetitive tasks and generating test scripts, AI is not only reducing costs but also enhancing the productivity of quality engineers.
Quality Engineering in Agile: A Shift Towards Integrated Teams
The growing importance of embedding QE into Agile teams is another major trend highlighted by the report. Currently, 40% of organizations have quality engineers integrated directly into their Agile workflows. This shift is a clear move away from traditional Testing Centers of Excellence (TCoEs), which have declined in use, now comprising only 27% of respondents’ QE structures, compared to a staggering 70% in previous years.
The focus on embedding QE within Agile teams ensures faster iterations and better alignment with business goals. Furthermore, cross-functional collaboration is recognized as critical for delivering higher-quality results, with 78% of respondents emphasizing its importance in ensuring better quality products faster.
Despite these advances, challenges remain. The report finds that 56% of organizations still view QE as a non-strategic function, and 53% acknowledge that their current QE processes are insufficient for Agile methodologies. This calls for a more significant focus on aligning QE metrics with broader business outcomes, such as customer satisfaction and revenue impact.
Data Quality: The Foundation for AI-Driven Testing
As organizations become more reliant on data-driven decision-making, the quality of their data takes on heightened importance. The report reveals that 64% of organizations now consider data quality a top priority, but many are still grappling with how to effectively manage it. Establishing clear ownership of data and improving frameworks for data governance are essential steps toward ensuring the accuracy and reliability of AI models used in QE.
Without high-quality data, AI’s ability to generate meaningful insights, create test scenarios, and predict outcomes is compromised. This explains why 58% of respondents rank data breaches as the most significant risk associated with Gen AI. As organizations integrate AI into their quality processes, ensuring robust data security becomes paramount.
Intelligent Product Validation: Testing Beyond Functionality
The validation of intelligent products is emerging as a critical component of modern QE practices. According to the report, 21% of testing budgets are now dedicated to validating smart technologies, reflecting the growing need for comprehensive strategies to ensure these products perform seamlessly in interconnected environments.
Functional correctness remains the top priority for validating intelligent products, with 30% of respondents citing it as the most important factor. However, security (23%) and data quality (21%) also rank highly, signaling a shift toward more holistic testing strategies that address the complexity of smart products.
The report also identifies challenges in testing these products, particularly when it comes to the validation of embedded AI models and the ability to test all integrations across devices and protocols. A lack of skilled testers further exacerbates these challenges, with 44% of organizations struggling to find talent capable of handling the intricacies of intelligent product testing.
Sustainability in Quality Engineering
With the rising concerns over climate change and environmental responsibility, 58% of organizations are prioritizing sustainability within their QE strategies. However, only 34% have implemented practices that measure the environmental impact of their testing activities. This highlights a significant gap between intent and execution, underscoring the need for more robust frameworks to track sustainability efforts.
Organizations are beginning to explore how QE can contribute to Green IT initiatives, with areas such as energy consumption monitoring, environmental data analysis, and optimization of test environments gaining traction. AI can play a pivotal role in these efforts, with 54% of respondents identifying energy efficiency optimization as one of the most valuable uses of AI in quality validation.
Key Recommendations for the Future
The report offers several key recommendations for organizations looking to stay competitive in the evolving QE landscape:
- Leverage Gen AI for Automation: Start experimenting with Gen AI to enhance and accelerate test automation processes. Gen AI’s potential extends beyond script generation, offering opportunities for self-adaptive automation systems that can boost both efficiency and effectiveness.
- Invest in QE Talent: To keep pace with AI and automation, organizations must invest in upskilling their quality engineers. Full-stack engineers, capable of working across the entire software lifecycle, are increasingly in demand.
- Focus on Business Performance Metrics: Shift away from traditional metrics like process efficiency and test coverage. Instead, focus on how QE initiatives contribute to business outcomes, such as customer satisfaction and revenue growth.
- Develop a Sustainability Strategy: Implement comprehensive processes to measure and reduce the environmental impact of QE activities. Integrating sustainability into testing will not only advance corporate social responsibility goals but also improve operational efficiency.
Conclusion
The World Quality Report 2024-25 paints a vivid picture of an industry on the cusp of transformation, driven by AI, automation, and sustainability. As organizations navigate this new landscape, adopting a forward-thinking approach to QE will be essential to gaining a competitive edge. By leveraging AI’s potential, investing in talent, and aligning quality initiatives with business goals, companies can ensure they are prepared for the challenges and opportunities that lie ahead.
The post AI and Automation Transforming Quality Engineering: Insights from the 2024 World Quality Report appeared first on Unite.AI.