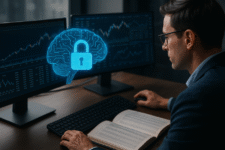
As artificial intelligence continues its rapid advance across industries, financial services firms find themselves at a crossroads. Eager to harness AI’s potential, yet wary of growing regulatory scrutiny, many institutions are discovering that the path to innovation is far more complex than anticipated. Recent headlines spotlight risks like AI hallucinations, model bias, and opaque decision-making—issues that regulators are increasingly keen to address.
Yet, behind the noise of generative AI hype and compliance concerns lies a more practical, overlooked opportunity. Success with AI doesn’t depend on building bigger models, but on providing them with the right and domain specific data to work effectively. Financial institutions sit on mountains of unstructured data trapped in contracts, statements, disclosures, emails, and legacy systems. Until that data is unlocked and made usable, AI will continue to fall short of its promise in the financial sector.
The Hidden Challenge: Trillions Locked in Unstructured Data
Financial institutions generate and manage staggering volumes of data daily. However, an estimated 80-90% of this data is unstructured, buried in contracts, emails, disclosures, reports, and communications. Unlike structured datasets neatly organized in databases, unstructured data is messy, varied, and difficult to process at scale using traditional methods.
This presents a critical challenge. AI systems are only as good as the data they’re fed. Without access to clean, contextual, and reliable information, even the most advanced models risk delivering inaccurate or misleading outputs. This is particularly problematic in financial services, where accuracy, transparency, and regulatory compliance are non-negotiable.
As firms race to adopt AI, many are discovering that their most valuable data assets remain trapped in outdated systems and siloed repositories. Unlocking this data is no longer a back-office concern—it’s central to AI success.
Regulatory Pressure and the Risk of Rushing AI
Regulators worldwide have begun sharpening their focus on AI use within financial services. Concerns over hallucinations and transparency, where AI models generate plausible but incorrect information without proper trackability, are mounting. Model bias and lack of explainability further complicate adoption, especially in areas like lending, risk assessment, and compliance, where opaque decisions can lead to legal exposure and reputational damage.
Surveys indicate that over 80% of financial institutions cite data reliability and explainability concerns as major factors slowing their AI initiatives. The fear of unintended consequences, coupled with tightening oversight, has created a cautious environment. Firms are under pressure to innovate, but wary of falling afoul of regulators or deploying AI systems that can’t be fully trusted.
In this climate, chasing generalized AI solutions or experimenting with off-the-shelf LLMs often leads to stalled projects, wasted investments, or worse—systems that amplify risk rather than mitigate it.
A Shift Toward Domain-Specific, Data-Centric AI
The breakthrough the industry needs isn’t another model. It’s a shift in focus, from model-building to data mastery. Domain-specific, unstructured data processing offers a more grounded approach to AI in financial services. Instead of relying on generic models trained on broad internet data, this method emphasizes extracting, structuring, and contextualizing the unique data that financial institutions already possess.
By leveraging AI designed to understand the nuances of financial language, documentation, and workflows, firms can turn previously inaccessible data into actionable intelligence. This enables automation, insights, and decision support rooted in the institution’s own trusted information, not external datasets prone to inaccuracies or irrelevance.
This approach delivers immediate ROI by improving efficiency and reducing risk, while also meeting regulatory expectations. By building systems with clear and traceable data pipelines, organizations gain the transparency and explainability needed to overcome two of the biggest challenges in AI adoption today
AI is Driving Real Results in the Financial World
While much of the AI conversation remains fixated on flashy innovations, domain-specific unstructured data processing is already transforming operations behind the scenes at some of the world’s largest banks and financial institutions. These organizations are using AI not to replace human expertise, but to augment it, automating the extraction of critical terms from contracts, flagging compliance risks buried in disclosures, or streamlining client communications analysis.
For example, a fundamental analysis of financial statements is a core function across financial services, but analysts often spend countless hours navigating the variability of each statement and deciphering the auditor’s notes. Firms leveraging AI solutions like ours have reduced processing times by 60%, allowing teams to shift their focus from manual review to strategic decision-making.
The impact is tangible. Manual processes that once took days or weeks are now completed in minutes. Risk management teams gain earlier visibility into potential issues. Compliance departments can respond faster and with greater confidence during audits or regulatory reviews. These AI implementations don’t require firms to gamble on unproven models. They build on existing data foundations, enhancing what’s already there.
This practical application of AI stands in stark contrast to the trial-and-error methods common in many generative AI projects. Rather than chasing the latest technology trends, it focuses on solving real business problems with accuracy and purpose.
De-Risking AI: What CTOs and Regulators Are Overlooking
In the rush to adopt AI, many financial services leaders—and even regulators—may be focusing too much on the model layer and not enough on the data layer. The allure of advanced algorithms often overshadows the fundamental truth that AI outcomes are dictated by data quality, relevance, and structure.
By prioritizing domain-specific data processing, institutions can de-risk AI initiatives from the start. This means investing in technologies and frameworks that can intelligently process unstructured data within the context of financial services, ensuring that outputs are not only accurate but also explainable and auditable.
This approach also positions firms to scale AI more effectively. Once unstructured data is transformed into usable formats, it becomes a foundation upon which multiple AI use cases can be built, whether for regulatory reporting, customer service automation, fraud detection, or investment analysis.Rather than treating each AI project as a standalone effort, mastering unstructured data creates a reusable asset, accelerating future innovation while maintaining control and compliance.
Moving Beyond the Hype Cycle
The financial services industry is at a pivotal moment. AI offers enormous potential, but realizing that potential requires a disciplined, data-first mindset. The current focus on hallucination risks and model bias, while valid, can distract from the more pressing issue: without unlocking and structuring the vast reserves of unstructured data, AI initiatives will continue to underdeliver.
Domain-specific unstructured data processing represents the kind of breakthrough that doesn’t make sensational headlines, but drives measurable, sustainable impact. It’s a reminder that in highly regulated, data-intensive industries like financial services, practical AI isn’t about chasing the next big thing. It’s about making better use of what’s already there.
As regulators continue to tighten oversight and firms look to balance innovation with risk management, those who focus on data mastery will be best positioned to lead. The future of AI in financial services won’t be defined by who has the flashiest model, but by who can unlock their data, deploy AI responsibly, and deliver consistent value in a complex, compliance-driven world.
The post AI’s Biggest Opportunity in Finance Isn’t New Models—It’s Unlocking Old Data appeared first on Unite.AI.