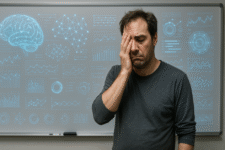
We’ve seen this story before: disruptive technology captures the imagination of business leaders across industries, promising transformation at scale. In the early 2010s, it was robotic process automation (RPA). Soon after, cloud computing took its turn. Today, generative AI (Gen AI) holds the spotlight – and organizations are diving headfirst into pilots without a clear path forward.
The result? A rising wave of what can be referred to as Generative AI Pilot Fatigue. It’s the state of exhaustion, frustration, and dwindling momentum that sets in when too many AI initiatives are launched without structure, purpose, or measurable goals. Companies run dozens of pilots simultaneously, often with overlapping intent but no clear success criteria. They chase potential across departments, but instead of unlocking efficiency or ROI, they create confusion, redundancy, and stalled innovation.
Defining Gen AI Pilot Fatigue
Generative AI pilot fatigue reflects a broader organizational challenge: infinite ambition without finite structure. The root causes are familiar to anyone who’s witnessed past technology waves:
- Infinite possibilities: Gen AI can be applied across every function – marketing, operations, HR, finance – which makes it tempting to launch multiple use cases without clear boundaries.
- Ease of deployment: Tools like OpenAI’s GPT models and Google’s Gemini allow teams to spin up pilots quickly with no engineering dependency – sometimes in a matter of hours.
- Lacking a sustainment plan: Gen AI requires good quality data to be effective. In many cases, data can become stale without implementing a process to ensure the data remains correct and current.
- Poor measurability: Unlike traditional IT deployments, it’s difficult to determine when a Gen AI tool is “good enough” to move from pilot to production. ROI is often murky or delayed.
- Integration hurdles: Many organizations struggle to plug Gen AI tools into existing systems, data pipelines, or workflows, adding time, complexity, and frustration.
- High resource demand: Pilots often require significant time, money, and human investment – especially around training and maintaining clean, usable data sets.
In short, Gen AI fatigue arises when experimentation outpaces strategy.
Why does this keep happening?
In many cases, it’s because organizations skip the foundational work. Before deploying any advanced tech, you must first optimize the processes you’re trying to improve. At Accruent, we’ve seen that just by streamlining workflows and ensuring data quality, companies can drive up to 50% efficiency gains before introducing AI at all. Layer Gen AI on top of a well-tuned system, and the improvement can double. But without that groundwork, even the most impressive AI models won’t deliver meaningful value.
Another pitfall is the absence of clear guardrails. Gen AI pilots shouldn’t be treated as infinite experiments. Success should be measured in defined outcomes – time saved, cost reduced, or capabilities expanded. There must be gates in place to advance, pivot, or end projects based on data-driven evaluation. Half of all Gen AI ideas may ultimately prove to be better suited for other technologies like RPA or no-code tools – and that’s okay. The goal isn’t to implement AI for the sake of implementing AI, but to solve business problems effectively.
Lessons from RPA and Cloud Migration
This isn’t the first time organizations have been swept up by tech enthusiasm. RPA promised to eliminate repetitive tasks; cloud migration promised flexibility and scale. Both delivered – eventually – but only for those who applied discipline to deployment.
One major takeaway? Don’t skip the foundation. We’ve seen firsthand that organizations can drive up to 50% efficiency gains just by streamlining existing workflows and improving data hygiene before introducing AI. When AI is applied to an optimized system, gains can double. But when AI is layered on top of broken processes, the impact is negligible.
The same is true for data. Gen AI models are only as good as the data they consume. Dirty, outdated, or inconsistent data will lead to poor outcomes – or worse, biased and misleading ones. That’s why companies must invest in robust data governance frameworks, a view supported by industry experts and emphasized in reports by McKinsey.
The Temptation of “Easy” AI
One of the double-edged swords of generative AI is its low barrier to entry. With pre-built models and user-friendly interfaces, anyone in an organization can spin up a pilot in a matter of days – sometimes hours or even minutes. While this accessibility is powerful, it also opens floodgates. Suddenly, you have teams across departments experimenting in silos, with little oversight or coordination. It’s not unusual to see dozens of Gen AI initiatives running simultaneously, each with different stakeholders, datasets, and definitions of success or lack thereof .
This fragmented approach leads to fatigue – not just from a resourcing standpoint, but from the growing frustration of not seeing tangible returns. Without centralized governance and a clear vision, even the most promising use cases can end up stuck in endless loops of iteration, refinement, and reevaluation.
Break the Cycle: Build with Intention
Start with treating Gen AI like any other enterprise technology investment – grounded in strategy, governance, and process optimization. Here are a few principles I’ve found critical:
- Start with the problem, not the tech. Too often, organizations chase Gen AI use cases because they’re exciting – not because they solve a defined business challenge. Begin by identifying friction points or inefficiencies in your workflows, and then ask: is Gen AI the best tool for the job?
- Optimize before you innovate. Before layering AI onto a broken process, fix the process. Streamlining operations can unlock major gains on their own – and makes it far easier to measure the additive impact of AI. As Bain & Company noted in a recent report, businesses that focus on foundational readiness see faster time to value from Gen AI.
- Validate your data. Ensure your models are trained on accurate, relevant, and ethically sourced data. Poor data quality is one of the top reasons pilots fail to scale, according to Gartner.
- Define what “good” looks like. Every pilot should have clear KPIs tied to business goals. Whether its reducing time spent on routine tasks or cutting operational costs, success must be measurable – and pilots must have decision gates to continue, pivot, or sunset.
- Keep a broad toolkit. Gen AI isn’t the answer to every problem. In some cases, automation via RPA, low-code apps, or machine learning might be faster, cheaper, or more sustainable. Be willing to say no to AI if the ROI doesn’t pencil out.
Looking Ahead: What Will Help vs What Might Hurt
In the coming years, pilot fatigue may get worse before it gets better. The pace of innovation is only accelerating, especially with emerging technologies like Agentic AI. The pressure to “do something with AI” is immense – and without the right guardrails, organizations risk being overwhelmed by the sheer volume of possibilities.
However, there’s reason for optimism. Development practices are maturing. Teams are beginning to treat Gen AI with the same rigor they apply to traditional software projects. We’re also seeing improvements in tooling. Advances in AI integration platforms and API orchestration are making it easier to slot Gen AI into existing tech stacks. Pre-trained models from providers like OpenAI, Meta, and Mistral reduce the burden on internal teams. And frameworks around ethical and responsible AI, like those championed by the AI Now Institute, are helping reduce ambiguity and risk. Perhaps most importantly, we’re seeing a rise in cross-functional AI literacy – a growing understanding among business and technical leaders alike about what AI can (and can’t) do.
Final Thought: It’s About Purpose, Not Pilots
At the end of the day, AI success comes down to intent. Generative AI has the potential to drive massive efficiency gains, unlock new capabilities, and transform industries – but only if it’s guided by strategy, supported by clean data, and measured by outcomes.
Without those anchors, it’s just another tech fad destined to exhaust your teams and disappoint your board.
If you want to avoid Gen AI pilot fatigue, don’t start with the technology. Start with a purpose. And build from there.
The post Avoiding Gen AI Pilot Fatigue: Leading with Purpose appeared first on Unite.AI.