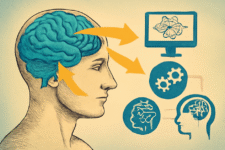
For centuries, human thinking has been understood through the lens of logic and reason. Traditionally, people have been seen as rational beings who use logic and deduction to understand the world. However, Geoffrey Hinton, a leading figure in Artificial Intelligence (AI), challenges this long-held belief. Hinton argues that humans are not purely rational but rather analogy machines, primarily relying on analogies to make sense of the world. This perspective changes our understanding of how human cognition works.
As AI continues to evolve, Hinton’s theory becomes increasingly relevant. By recognizing that humans think in analogies rather than pure logic, AI can be developed to mimic better how we naturally process information. This transformation not only alters our understanding of the human mind but also carries significant implications for the future of AI development and its role in daily life.
Understanding Hinton’s Analogy Machine Theory
Geoffrey Hinton’s analogy machine theory presents a fundamental rethinking of human cognition. According to Hinton, the human brain operates primarily through analogy, not through rigid logic or reasoning. Instead of relying on formal deduction, humans navigate the world by recognizing patterns from past experiences and applying them to new situations. This analogy-based thinking is the foundation of many cognitive processes, including decision-making, problem-solving, and creativity. While reasoning does play a role, it is a secondary process that only comes into play when precision is required, such as in mathematical problems.
Neuroscientific research backs up this theory, showing that the brain’s structure is optimized for recognizing patterns and drawing analogies rather than being a center for pure logical processing. Functional magnetic resonance imaging (fMRI) studies show that areas of the brain associated with memory and associative thinking are activated when people engage in tasks involving analogy or pattern recognition. This makes sense from an evolutionary perspective, as analogical thinking allows humans to quickly adapt to new environments by recognizing familiar patterns, thus helping in fast decision-making.
Hinton’s theory contrasts with traditional cognitive models that have long emphasized logic and reasoning as the central processes behind human thought. For much of the 20th century, scientists viewed the brain as a processor that applied deductive reasoning to draw conclusions. This perspective did not account for the creativity, flexibility, and fluidity of human thinking. Hinton’s analogy machine theory, on the other hand, argues that our primary method of understanding the world involves drawing analogies from a wide range of experiences. Reasoning, while important, is secondary and only comes into play in specific contexts, such as in mathematics or problem-solving.
This rethinking of cognition is not unlike the revolutionary impact psychoanalysis had in the early 20th century. Just as psychoanalysis uncovered unconscious motivations driving human behavior, Hinton’s analogy machine theory reveals how the mind processes information through analogies. It challenges the idea that human intelligence is primarily rational, instead suggesting that we are pattern-based thinkers, using analogies to make sense of the world around us.
How Analogical Thinking Shapes AI Development
Geoffrey Hinton’s analogy machine theory not only reshapes our understanding of human cognition but also has profound implications for the development of AI. Modern AI systems, especially Large Language Models (LLMs) like GPT-4, are starting to adopt a more human-like approach to problem-solving. Rather than relying solely on logic, these systems now use vast amounts of data to recognize patterns and apply analogies, closely mimicking how humans think. This method enables AI to process complex tasks like natural language understanding and image recognition in a way that aligns with the analogy-based thinking Hinton describes.
The growing connection between human thinking and AI learning is becoming clearer as technology advances. Earlier AI models were built on strict rule-based algorithms that followed logical patterns to generate outputs. However, today’s AI systems, like GPT-4, work by identifying patterns and drawing analogies, much like how humans use their past experiences to understand new situations. This change in approach brings AI closer to human-like reasoning, where analogies, rather than just logical deductions, guide actions and decisions.
With the ongoing developments of AI systems, Hinton’s work is influencing the direction of future AI architectures. His research, particularly on the GLOM (Global Linear and Output Models) project, is exploring how AI can be designed to incorporate analogical reasoning more deeply. The goal is to develop systems that can think intuitively, much like humans do when making connections across various ideas and experiences. This could lead to more adaptable, flexible AI that does not just solve problems but does so in a way that mirrors human cognitive processes.
Philosophical and Societal Implications of Analogy-Based Cognition
As Geoffrey Hinton’s analogy machine theory gains attention, it brings with it profound philosophical and societal implications. Hinton’s theory challenges the long-standing belief that human cognition is primarily rational and based on logic. Instead, it suggests that humans are fundamentally analogy machines, using patterns and associations to navigate the world. This change in understanding could reshape disciplines like philosophy, psychology, and education, which have traditionally emphasized rational thought. Suppose creativity is not merely the result of novel combinations of ideas but rather the ability to make analogies between different domains. In that case, we may gain a new perspective on how creativity and innovation function.
This realization could have a significant impact on education. If humans primarily rely on analogical thinking, education systems may need to adjust by focusing less on pure logical reasoning and more on enhancing students’ ability to recognize patterns and make connections across different fields. This approach would cultivate productive intuition, helping students solve problems by applying analogies to new and complex situations, ultimately enhancing their creativity and problem-solving skills.
As AI systems evolve, there is growing potential for them to mirror human cognition by adopting analogy-based reasoning. If AI systems develop the ability to recognize and apply analogies in a similar way to humans, it could transform how they approach decision-making. However, this advancement brings important ethical considerations. With AI potentially surpassing human capabilities in drawing analogies, questions will arise about their role in decision-making processes. Ensuring these systems are used responsibly, with human oversight, will be critical to prevent misuse or unintended consequences.
While Geoffrey Hinton’s analogy machine theory presents a fascinating new perspective on human cognition, some concerns need to be addressed. One concern, based on the Chinese Room argument, is that while AI can recognize patterns and make analogies, it may not truly understand the meaning behind them. This raises questions about the depth of understanding AI can achieve.
Additionally, the reliance on analogy-based thinking may not be as effective in fields like mathematics or physics, where precise logical reasoning is essential. There are also concerns that cultural differences in how analogies are made could limit the universal application of Hinton’s theory across different contexts.
The Bottom Line
Geoffrey Hinton’s analogy machine theory provides a groundbreaking perspective on human cognition, highlighting how our minds rely more on analogies than pure logic. This not only reshapes the study of human intelligence but also opens new possibilities for AI development.
By designing AI systems that mimic human analogy-based reasoning, we can create machines that process information in ways that are more natural and intuitive. However, as AI evolves to adopt this approach, there are important ethical and practical considerations, such as ensuring human oversight and addressing concerns about AI’s depth of understanding. Ultimately, embracing this new model of thinking could redefine creativity, learning, and the future of AI, promoting smarter and more adaptable technologies.
The post Beyond Logic: Rethinking Human Thought with Geoffrey Hinton’s Analogy Machine Theory appeared first on Unite.AI.