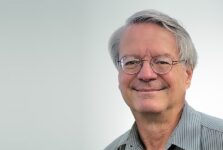
David Maher serves as Intertrust’s Executive Vice President and Chief Technology Officer. With over 30 years of experience in trusted distributed systems, secure systems, and risk management Dave has led R&D efforts and held key leadership positions across the company’s subsidiaries. He was past president of Seacert Corporation, a Certificate Authority for digital media and IoT, and President of whiteCryption Corporation, a developer of systems for software self-defense. He also served as co-chairman of the Marlin Trust Management Organization (MTMO), which oversees the world’s only independent digital rights management ecosystem.
Intertrust developed innovations enabling distributed operating systems to secure and govern data and computations over open networks, resulting in a foundational patent on trusted distributed computing.
Originally rooted in research, Intertrust has evolved into a product-focused company offering trusted computing services that unify device and data operations, particularly for IoT and AI. Its markets include media distribution, device identity/authentication, digital energy management, analytics, and cloud storage security.
How can we close the AI trust gap and address the public’s growing concerns about AI safety and reliability?
Transparency is the most important quality that I believe will help address the growing concerns about AI. Transparency includes features that help both consumers and technologists understand what AI mechanisms are part of systems we interact with, what kind of pedigree they have: how an AI model is trained, what guardrails exist, what policies were applied in the model development, and what other assurances exist for a given mechanism’s safety and security. With greater transparency, we will be able to address real risks and issues and not be distracted as much by irrational fears and conjectures.
What role does metadata authentication play in ensuring the trustworthiness of AI outputs?
Metadata authentication helps increase our confidence that assurances about an AI model or other mechanism are reliable. An AI model card is an example of a collection of metadata that can assist in evaluating the use of an AI mechanism (model, agent, etc.) for a specific purpose. We need to establish standards for clarity and completeness for model cards with standards for quantitative measurements and authenticated assertions about performance, bias, properties of training data, etc.
How can organizations mitigate the risk of AI bias and hallucinations in large language models (LLMs)?
Red teaming is a general approach to addressing these and other risks during the development and pre-release of models. Originally used to evaluate secure systems, the approach is now becoming standard for AI-based systems. It is a systems approach to risk management that can and should include the entire life cycle of a system from initial development to field deployment, covering the entire development supply chain. Especially critical is the classification and authentication of the training data used for a model.
What steps can companies take to create transparency in AI systems and reduce the risks associated with the “black box” problem?
Understand how the company is going to use the model and what kinds of liabilities it may have in deployment, whether for internal use or use by customers, either directly or indirectly. Then, understand what I call the pedigrees of the AI mechanisms to be deployed, including assertions on a model card, results of red-team trials, differential analysis on the company’s specific use, what has been formally evaluated, and what have been other people’s experience. Internal testing using a comprehensive test plan in a realistic environment is absolutely required. Best practices are evolving in this nascent area, so it is important to keep up.
How can AI systems be designed with ethical guidelines in mind, and what are the challenges in achieving this across different industries?
This is an area of research, and many claim that the notion of ethics and the current versions of AI are incongruous since ethics are conceptually based, and AI mechanisms are mostly data-driven. For example, simple rules that humans understand, like “don’t cheat,” are difficult to ensure. However, careful analysis of interactions and conflicts of goals in goal-based learning, exclusion of sketchy data and disinformation, and building in rules that require the use of output filters that enforce guardrails and test for violations of ethical principles such as advocating or sympathizing with the use of violence in output content should be considered. Similarly, rigorous testing for bias can help align a model more with ethical principles. Again, much of this can be conceptual, so care must be given to test the effects of a given approach since the AI mechanism will not “understand” instructions the way humans do.
What are the key risks and challenges that AI faces in the future, especially as it integrates more with IoT systems?
We want to use AI to automate systems that optimize critical infrastructure processes. For example, we know that we can optimize energy distribution and use using virtual power plants, which coordinate thousands of elements of energy production, storage, and use. This is only practical with massive automation and the use of AI to aid in minute decision-making. Systems will include agents with conflicting optimization objectives (say, for the benefit of the consumer vs the supplier). AI safety and security will be critical in the widescale deployment of such systems.
What type of infrastructure is needed to securely identify and authenticate entities in AI systems?
We will require a robust and efficient infrastructure whereby entities involved in evaluating all aspects of AI systems and their deployment can publish authoritative and authentic claims about AI systems, their pedigree, available training data, the provenance of sensor data, security affecting incidents and events, etc. That infrastructure will also need to make it efficient to verify claims and assertions by users of systems that include AI mechanisms and by elements within automated systems that make decisions based on outputs from AI models and optimizers.
Could you share with us some insights into what you are working on at Intertrust and how it factors into what we have discussed?
We research and design technology that can provide the kind of trust management infrastructure that is required in the previous question. We are specifically addressing issues of scale, latency, security and interoperability that arise in IoT systems that include AI components.
How does Intertrust’s PKI (Public Key Infrastructure) service secure IoT devices, and what makes it scalable for large-scale deployments?
Our PKI was designed specifically for trust management for systems that include the governance of devices and digital content. We have deployed billions of cryptographic keys and certificates that assure compliance. Our current research addresses the scale and assurances that massive industrial automation and critical worldwide infrastructure require, including best practices for “zero-trust” deployments and device and data authentication that can accommodate trillions of sensors and event generators.
What motivated you to join NIST’s AI initiatives, and how does your involvement contribute to developing trustworthy and safe AI standards?
NIST has tremendous experience and success in developing standards and best practices in secure systems. As a Principal Investigator for the US AISIC from Intertrust, I can advocate for important standards and best practices in developing trust management systems that include AI mechanisms. From past experience, I particularly appreciate the approach that NIST takes to promote creativity, progress, and industrial cooperation while helping to formulate and promulgate important technical standards that promote interoperability. These standards can spur the adoption of beneficial technologies while addressing the kinds of risks that society faces.
Thank you for the great interview, readers who wish to learn more should visit Intertrust.
The post David Maher, CTO of Intertrust – Interview Series appeared first on Unite.AI.