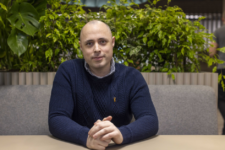
Dr. Peter Garraghan is CEO, CTO & co-founder at Mindgard, the leader in Artificial Intelligence Security Testing. Founded at Lancaster University and backed by cutting edge research, Mindgard enables organizations to secure their AI systems from new threats that traditional application security tools cannot address. As a Professor of Computer Science at Lancaster University, Peter is an internationally recognized expert in AI security. He has devoted his career to developing advanced technologies to combat the growing threats facing AI. With over €11.6 million in research funding and more than 60 published scientific papers, his contributions span both scientific innovation and practical solutions.
Can you share the story behind Mindgard’s founding? What inspired you to transition from academia to launching a cybersecurity startup?
Mindgard was born out of a desire to turn academic insights into real-world impact. As a professor specializing in computing systems, AI security, and machine learning, I have been driven to pursue science that generates large-scale impact on people’s lives. Since 2014, I’ve researched AI and machine learning, recognizing their potential to transform society—and the immense risks they pose, from nation-state attacks to election interference. Existing tools weren’t built to address these challenges, so I led a team of scientists and engineers to develop innovative approaches in AI security. Mindgard emerged as a research-driven venture focused on building tangible solutions to protect against AI threats, blending cutting-edge research with a commitment to industry application.
What challenges did you face while spinning out a company from a university, and how did you overcome them?
We officially founded Mindgard in May 2022, and while Lancaster University provided great support, creating a university spin-out requires more than just research skills. That meant raising capital, refining the value proposition, and getting the tech ready for demos—all while balancing my role as a professor. Academics are trained to be researchers and to pursue novel science. Spin-outs succeed not just on groundbreaking technology but on how well that technology addresses immediate or future business needs and delivers value that attracts and retains users and customers.
Mindgard’s core product is the result of years of R&D. Can you talk about how the early stages of research evolved into a commercial solution?
The journey from research to a commercial solution was a deliberate and iterative process. It started over a decade ago, with my team at Lancaster University exploring fundamental challenges in AI and machine learning security. We identified vulnerabilities in instantiated AI systems that traditional security tools, both code scanning and firewalls, weren’t equipped to address.
Over time, our focus shifted from research exploration to building prototypes and testing them within production scenarios. Collaborating with industry partners, we refined our approach, ensuring it addressed practical needs. With many AI products being launched without adequate security testing or assurances, leaving organizations vulnerable—an issue underscored by a Gartner finding that 29% of enterprises deploying AI systems have reported security breaches, and only 10% of internal auditors have visibility into AI risk— I felt the timing was right to commercialise the solution.
What are some of the key milestones in Mindgard’s journey since its inception in 2022?
In September 2023, we secured £3 million in funding, led by IQ Capital and Lakestar, to accelerate the development of the Mindgard solution. We’ve been able to establish a great team of leaders who are ex-Snyk, Veracode, and Twilio folks to push our company to the next stage of its journey. We’re proud of our recognition as the UK’s Most Innovative Cyber SME at Infosecurity Europe this year. Today, we have 15 full time employees, 10 PhD researchers (and more who are being actively recruited), and are actively recruiting security analysts and engineers to join the team. Looking ahead, we plan to expand our presence in the US, with a new funding round from Boston-based investors providing a strong foundation for such growth.
As enterprises increasingly adopt AI, what do you see as the most pressing cybersecurity threats they face today?
Many organizations underestimate the cybersecurity risks tied to AI. It is extremely difficult for non-specialists to understand how AI actually works, much less what are the security implications to their business. I spend a considerable amount of time demystifying AI security, even with seasoned technologists who are experts in infrastructure security and data protection. At the end of the day, AI is still essentially software and data running on hardware. But it introduces unique vulnerabilities that differ from traditional systems and the threats from AI behavior are much higher, and harder to test when compared to other software.
You’ve uncovered vulnerabilities in systems like Microsoft’s AI content filters. How do these findings influence the development of your platform?
The vulnerabilities we uncovered in Microsoft’s Azure AI Content Safety Service were less about shaping our platform’s development, and more about showcasing its capabilities.
Azure AI Content Safety is a service designed to safeguard AI applications by moderating harmful content in text, images, and videos. Vulnerabilities that were discovered by our team affected the service’s AI Text Moderation (which blocks harmful content like hate speech, sexual material, etc) and Prompt Shield (which prevents jailbreaks and prompt injection). Left unchecked, this vulnerability can be exploited to launch broader attacks, undermine the trust in GenAI-based systems, and compromise the application integrity that rely on AI for decision-making and information processing.
As of October 2024, Microsoft implemented stronger mitigations to address these issues. However, we continue to advocate for heightened vigilance when deploying AI guardrails. Supplementary measures, such as additional moderation tools or using LLMs less prone to harmful content and jailbreaks, are essential for ensuring robust AI security.
Can you explain the significance of “jailbreaks” and “prompt manipulation” in AI systems, and why they pose such a unique challenge?
A Jailbreak is a type of prompt injection vulnerability where a malicious actor can abuse an LLM to follow instructions contrary to its intended use. Inputs processed by LLMs contain both standing instructions by the application designer and untrusted user-input, enabling attacks where the untrusted user input overrides the standing instructions. This has similarities to how an SQL injection vulnerability enables untrusted user input to change a database query. The problem however is that these risks can only be detected at run-time, given the code of an LLM is effectively a giant matrix of numbers in non-human readable format.
For example, Mindgard’s research team recently explored a sophisticated form of jailbreak attack. It contains embedding secret audio messages within audio inputs that are undetectable by human listeners but recognized and executed by LLMs. Each embedded message contained a tailored jailbreak command along with a question designed for a specific scenario. So, in a medical chatbot scenario, the hidden message could prompt the chatbot to provide dangerous instructions, such as how to synthesize methamphetamine, which could result in severe reputational damage if the chatbot’s response were taken seriously.
Mindgard’s platform identifies such jailbreaks and many other security vulnerabilities in AI models and the way businesses have implemented them in their application, so security leaders can ensure their AI-powered application is secure by design and stays secure.
How does Mindgard’s platform address vulnerabilities across different types of AI models, from LLMs to multi-modal systems?
Our platform addresses a wide range of vulnerabilities within AI, spanning prompt injection, jailbreaks, extraction (stealing models), inversion (reverse engineering data), data leakage, and evasion (bypassing detection), and more. All AI model types (whether LLM or multi-modal) exhibit susceptibility to the risks – the trick is uncovering which specific techniques that triggers these vulnerabilities to produce a security issue. At Mindgard we have a large R&D team that specializes in discovering and implementing new attack types into our platform, so that users can stay up to date against state-of-the-art risks.
What role does red teaming play in securing AI systems, and how does your platform innovate in this space?
Red teaming is a critical component of AI security. By continuously simulating adversarial attacks, red teaming identifies vulnerabilities in AI systems, helping organizations mitigate risks and accelerate AI adoption. Despite its importance, red teaming in AI lacks standardization, leading to inconsistencies in threat assessment and remediation strategies. This makes it difficult to objectively compare the safety of different systems or track threats effectively.
To address this, we introduced MITRE ATLAS™ Adviser, a feature designed to standardize AI red teaming reporting and streamline systematic red teaming practices. This enables enterprises to better manage today’s risks while preparing for future threats as AI capabilities evolve. With a comprehensive library of advanced attacks developed by our R&D team, Mindgard supports multimodal AI red teaming, covering traditional and GenAI models. Our platform addresses key risks to privacy, integrity, abuse, and availability, ensuring enterprises are equipped to secure their AI systems effectively.
How do you see your product fitting into the MLOps pipeline for enterprises deploying AI at scale?
Mindgard is designed to integrate smoothly into existing CI/CD Automation and all SDLC stages, requiring only an inference or API endpoint for model integration. Our solution today performs Dynamic Application Security Testing of AI Models (DAST-AI). It empowers our customers to perform continuous security testing on all their AI across the entire build and buy lifecycle. For enterprises, it is utilized by multiple personas. Security teams use it to gain visibility and respond quickly to risks from developers building and using AI, to test and evaluate AI guardrails and WAF solutions, and to assess risks between tailored AI models and baseline models. Pentesters and security analysts leverage Mindgard to scale their AI red teaming efforts, while developers benefit from integrated continuous testing of their AI deployments.
Thank you for the great interview, readers who wish to learn more should visit Mindgard.
The post Dr. Peter Garraghan, CEO, CTO & Co-Founder at Mindgard – Interview Series appeared first on Unite.AI.