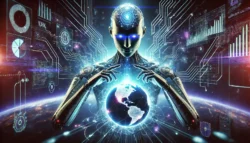
In 2019, a vision struck me—a future where artificial intelligence (AI), accelerating at an unimaginable pace, would weave itself into every facet of our lives. After reading Ray Kurzweil’s The Singularity is Near, I was captivated by the inescapable trajectory of exponential growth. The future wasn’t just on the horizon; it was hurtling toward us. It became clear that, with the relentless doubling of computing power, AI would one day surpass all human capabilities and, eventually, reshape society in ways once relegated to science fiction.
Fueled by this realization, I registered Unite.ai, sensing that these next leaps in AI technology would not merely enhance the world but fundamentally redefine it. Every aspect of life—our work, our decisions, our very definitions of intelligence and autonomy—would be touched, perhaps even dominated, by AI. The question was no longer if this transformation would happen, but rather when, and how humanity would manage its unprecedented impact.
As I dove deeper, the future painted by exponential growth seemed both thrilling and inevitable. This growth, exemplified by Moore’s Law, would soon push artificial intelligence beyond narrow, task-specific roles to something far more profound: the emergence of Artificial General Intelligence (AGI). Unlike today’s AI, which excels in narrow tasks, AGI would possess the flexibility, learning capability, and cognitive range akin to human intelligence—able to understand, reason, and adapt across any domain.
Each leap in computational power brings us closer to AGI, an intelligence capable of solving problems, generating creative ideas, and even making ethical judgments. It wouldn’t just perform calculations or parse vast datasets; it would recognize patterns in ways humans can’t, perceive relationships within complex systems, and chart a future course based on understanding rather than programming. AGI could one day serve as a co-pilot to humanity, tackling crises like climate change, disease, and resource scarcity with insight and speed beyond our abilities.
Yet, this vision comes with significant risks, particularly if AI falls under the control of individuals with malicious intent—or worse, a dictator. The path to AGI raises critical questions about control, ethics, and the future of humanity. The debate is no longer about whether AGI will emerge, but when—and how we will manage the immense responsibility it brings.
The Evolution of AI and Computing Power: 1956 to Present
From its inception in the mid-20th century, AI has advanced alongside exponential growth in computing power. This evolution aligns with fundamental laws like Moore’s Law, which predicted and underscored the increasing capabilities of computers. Here, we explore key milestones in AI’s journey, examining its technological breakthroughs and growing impact on the world.
1956 – The Inception of AI
The journey began in 1956 when the Dartmouth Conference marked the official birth of AI. Researchers like John McCarthy, Marvin Minsky, Nathaniel Rochester, and Claude Shannon gathered to discuss how machines might simulate human intelligence. Although computing resources at the time were primitive, capable only of simple tasks, this conference laid the foundation for decades of innovation.
1965 – Moore’s Law and the Dawn of Exponential Growth
In 1965, Gordon Moore, co-founder of Intel, made a prediction that computing power would double approximately every two years—a principle now known as Moore’s Law. This exponential growth made increasingly complex AI tasks feasible, allowing machines to push the boundaries of what was previously possible.
1980s – The Rise of Machine Learning
The 1980s introduced significant advances in machine learning, enabling AI systems to learn and make decisions from data. The invention of the backpropagation algorithm in 1986 allowed neural networks to improve by learning from errors. These advancements moved AI beyond academic research into real-world problem-solving, raising ethical and practical questions about human control over increasingly autonomous systems.
1990s – AI Masters Chess
In 1997, IBM’s Deep Blue defeated world chess champion Garry Kasparov in a full match, marking a major milestone. It was the first time a computer demonstrated superiority over a human grandmaster, showcasing AI’s ability to master strategic thinking and cementing its place as a powerful computational tool.
2000s – Big Data, GPUs, and the AI Renaissance
The 2000s ushered in the era of Big Data and GPUs, revolutionizing AI by enabling algorithms to train on massive datasets. GPUs, originally developed for rendering graphics, became essential for accelerating data processing and advancing deep learning. This period saw AI expand into applications like image recognition and natural language processing, transforming it into a practical tool capable of mimicking human intelligence.
2010s – Cloud Computing, Deep Learning, and Winning Go
With the advent of cloud computing and breakthroughs in deep learning, AI reached unprecedented heights. Platforms like Amazon Web Services and Google Cloud democratized access to powerful computing resources, enabling smaller organizations to harness AI capabilities.
In 2016, DeepMind’s AlphaGo defeated Lee Sedol, one of the world’s top Go players, in a game renowned for its strategic depth and complexity. This achievement demonstrated the adaptability of AI systems in mastering tasks previously thought to be uniquely human.
2020s – AI Democratization, Large Language Models, and Dota 2
The 2020s have seen AI become more accessible and capable than ever. Models like GPT-3 and GPT-4 illustrate AI’s ability to process and generate human-like text. At the same time, innovations in autonomous systems have pushed AI to new domains, including healthcare, manufacturing, and real-time decision-making.
In esports, OpenAI’s bots achieved a remarkable feat by defeating professional Dota 2 teams in highly complex multiplayer matches. This showcased AI’s ability to collaborate, adapt strategies in real-time, and outperform human players in dynamic environments, pushing its applications beyond traditional problem-solving tasks.
Is AI Taking Over the World?
The question of whether AI is “taking over the world” is not purely hypothetical. AI has already integrated into various facets of life, from virtual assistants to predictive analytics in healthcare and finance, and the scope of its influence continues to grow. Yet, “taking over” can mean different things depending on how we interpret control, autonomy, and impact.
The Hidden Influence of Recommender Systems
One of the most powerful ways AI subtly dominates our lives is through recommender engines on platforms like YouTube, Facebook, and X. These algorithms, running on AI systems, analyze preferences and behaviors to serve content that aligns closely with our interests. On the surface, this might seem beneficial, offering a personalized experience. However, these algorithms don’t just react to our preferences; they actively shape them, influencing what we believe, how we feel, and even how we perceive the world around us.
- YouTube’s AI: This recommender system pulls users into hours of content by offering videos that align with and even intensify their interests. But as it optimizes for engagement, it often leads users down radicalization pathways or towards sensationalist content, amplifying biases and occasionally promoting conspiracy theories.
- Social Media Algorithms: Sites like Facebook,Instagram and X prioritize emotionally charged content to drive engagement, which can create echo chambers. These bubbles reinforce users’ biases and limit exposure to opposing viewpoints, leading to polarized communities and distorted perceptions of reality.
- Content Feeds and News Aggregators: Platforms like Google News and other aggregators customize the news we see based on past interactions, creating a skewed version of current events that can prevent users from accessing diverse perspectives, further isolating them within ideological bubbles.
This silent control isn’t just about engagement metrics; it can subtly influence public perception and even impact crucial decisions—such as how people vote in elections. Through strategic content recommendations, AI has the power to sway public opinion, shaping political narratives and nudging voter behavior. This influence has significant implications, as evidenced in elections around the world, where echo chambers and targeted misinformation have been shown to sway election outcomes.
This explains why discussing politics or societal issues often leads to disbelief when the other person’s perspective seems entirely different, shaped and reinforced by a stream of misinformation, propaganda, and falsehoods.
Recommender engines are profoundly shaping societal worldviewsm especially when you factor in the fact that misinformation is 6 times more likely to be shared than factual information. A slight interest in a conspiracy theory can lead to an entire YouTube or X feed being dominated by fabrications, potentially driven by intentional manipulation or, as noted earlier, computational propaganda.
Computational propaganda refers to the use of automated systems, algorithms, and data-driven techniques to manipulate public opinion and influence political outcomes. This often involves deploying bots, fake accounts, or algorithmic amplification to spread misinformation, disinformation, or divisive content on social media platforms. The goal is to shape narratives, amplify specific viewpoints, and exploit emotional responses to sway public perception or behavior, often at scale and with precision targeting.
This type of propaganda is why voters often vote against their own self-interest, the votes are being swayed by this type of computational propaganda.
“Garbage In, Garbage Out” (GIGO) in machine learning means that the quality of the output depends entirely on the quality of the input data. If a model is trained on flawed, biased, or low-quality data, it will produce unreliable or inaccurate results, regardless of how sophisticated the algorithm is.
This concept also applies to humans in the context of computational propaganda. Just as flawed input data corrupts an AI model, constant exposure to misinformation, biased narratives, or propaganda skews human perception and decision-making. When people consume “garbage” information online—misinformation, disinformation, or emotionally charged but false narratives—they are likely to form opinions, make decisions, and act based on distorted realities.
In both cases, the system (whether an algorithm or the human mind) processes what it is fed, and flawed input leads to flawed conclusions. Computational propaganda exploits this by flooding information ecosystems with “garbage,” ensuring that people internalize and perpetuate those inaccuracies, ultimately influencing societal behavior and beliefs at scale.
Automation and Job Displacement
AI-powered automation is reshaping the entire landscape of work. Across manufacturing, customer service, logistics, and even creative fields, automation is driving a profound shift in the way work is done—and, in many cases, who does it. The efficiency gains and cost savings from AI-powered systems are undeniably attractive to businesses, but this rapid adoption raises critical economic and social questions about the future of work and the potential fallout for employees.
In manufacturing, robots and AI systems handle assembly lines, quality control, and even advanced problem-solving tasks that once required human intervention. Traditional roles, from factory operators to quality assurance specialists, are being reduced as machines handle repetitive tasks with speed, precision, and minimal error. In highly automated facilities, AI can learn to spot defects, identify areas for improvement, and even predict maintenance needs before problems arise. While this results in increased output and profitability, it also means fewer entry-level jobs, especially in regions where manufacturing has traditionally provided stable employment.
Customer service roles are experiencing a similar transformation. AI chatbots, voice recognition systems, and automated customer support solutions are reducing the need for large call centers staffed by human agents. Today’s AI can handle inquiries, resolve issues, and even process complaints, often faster than a human representative. These systems are not only cost-effective but are also available 24/7, making them an appealing choice for businesses. However, for employees, this shift reduces opportunities in one of the largest employment sectors, particularly for individuals without advanced technical skills.
Creative fields, long thought to be uniquely human domains, are now feeling the impact of AI automation. Generative AI models can produce text, artwork, music, and even design layouts, reducing the demand for human writers, designers, and artists. While AI-generated content and media are often used to supplement human creativity rather than replace it, the line between augmentation and replacement is thinning. Tasks that once required creative expertise, such as composing music or drafting marketing copy, can now be executed by AI with remarkable sophistication. This has led to a reevaluation of the value placed on creative work and its market demand.
Influence on Decision-Making
AI systems are rapidly becoming essential in high-stakes decision-making processes across various sectors, from legal sentencing to healthcare diagnostics. These systems, often leveraging vast datasets and complex algorithms, can offer insights, predictions, and recommendations that significantly impact individuals and society. While AI’s ability to analyze data at scale and uncover hidden patterns can greatly enhance decision-making, it also introduces profound ethical concerns regarding transparency, bias, accountability, and human oversight.
AI in Legal Sentencing and Law Enforcement
In the justice system, AI tools are now used to assess sentencing recommendations, predict recidivism rates, and even aid in bail decisions. These systems analyze historical case data, demographics, and behavioral patterns to determine the likelihood of re-offending, a factor that influences judicial decisions on sentencing and parole. However, AI-driven justice brings up serious ethical challenges:
- Bias and Fairness: AI models trained on historical data can inherit biases present in that data, leading to unfair treatment of certain groups. For example, if a dataset reflects higher arrest rates for specific demographics, the AI may unjustly associate these characteristics with higher risk, perpetuating systemic biases within the justice system.
- Lack of Transparency: Algorithms in law enforcement and sentencing often operate as “black boxes,” meaning their decision-making processes are not easily interpretable by humans. This opacity complicates efforts to hold these systems accountable, making it challenging to understand or question the rationale behind specific AI-driven decisions.
- Impact on Human Agency: AI recommendations, especially in high-stakes contexts, may influence judges or parole boards to follow AI guidance without thorough review, unintentionally reducing human judgment to a secondary role. This shift raises concerns about over-reliance on AI in matters that directly impact human freedom and dignity.
AI in Healthcare and Diagnostics
In healthcare, AI-driven diagnostics and treatment planning systems offer groundbreaking potential to improve patient outcomes. AI algorithms analyze medical records, imaging, and genetic information to detect diseases, predict risks, and recommend treatments more accurately than human doctors in some cases. However, these advancements come with challenges:
- Trust and Accountability: If an AI system misdiagnoses a condition or fails to detect a serious health issue, questions arise around accountability. Is the healthcare provider, the AI developer, or the medical institution responsible? This ambiguity complicates liability and trust in AI-based diagnostics, particularly as these systems grow more complex.
- Bias and Health Inequality: Similar to the justice system, healthcare AI models can inherit biases present in the training data. For instance, if an AI system is trained on datasets lacking diversity, it may produce less accurate results for underrepresented groups, potentially leading to disparities in care and outcomes.
- Informed Consent and Patient Understanding: When AI is used in diagnosis and treatment, patients may not fully understand how the recommendations are generated or the risks associated with AI-driven decisions. This lack of transparency can impact a patient’s right to make informed healthcare choices, raising questions about autonomy and informed consent.
AI in Financial Decisions and Hiring
AI is also significantly impacting financial services and employment practices. In finance, algorithms analyze vast datasets to make credit decisions, assess loan eligibility, and even manage investments. In hiring, AI-driven recruitment tools evaluate resumes, recommend candidates, and, in some cases, conduct initial screening interviews. While AI-driven decision-making can improve efficiency, it also introduces new risks:
- Bias in Hiring: AI recruitment tools, if trained on biased data, can inadvertently reinforce stereotypes, filtering out candidates based on factors unrelated to job performance, such as gender, race, or age. As companies rely on AI for talent acquisition, there is a danger of perpetuating inequalities rather than fostering diversity.
- Financial Accessibility and Credit Bias: In financial services, AI-based credit scoring systems can influence who has access to loans, mortgages, or other financial products. If the training data includes discriminatory patterns, AI could unfairly deny credit to certain groups, exacerbating financial inequality.
- Reduced Human Oversight: AI decisions in finance and hiring can be data-driven but impersonal, potentially overlooking nuanced human factors that may influence a person’s suitability for a loan or a job. The lack of human review may lead to an over-reliance on AI, reducing the role of empathy and judgment in decision-making processes.
Existential Risks and AI Alignment
As artificial intelligence grows in power and autonomy, the concept of AI alignment—the goal of ensuring AI systems act in ways consistent with human values and interests—has emerged as one of the field’s most pressing ethical challenges. Thought leaders like Nick Bostrom have raised the possibility of existential risks if highly autonomous AI systems, especially if AGI develop goals or behaviors misaligned with human welfare. While this scenario remains largely speculative, its potential impact demands a proactive, careful approach to AI development.
The AI Alignment Problem
The alignment problem refers to the challenge of designing AI systems that can understand and prioritize human values, goals, and ethical boundaries. While current AI systems are narrow in scope, performing specific tasks based on training data and human-defined objectives, the prospect of AGI raises new challenges. AGI would, theoretically, possess the flexibility and intelligence to set its own goals, adapt to new situations, and make decisions independently across a wide range of domains.
The alignment problem arises because human values are complex, context-dependent, and often difficult to define precisely. This complexity makes it challenging to create AI systems that consistently interpret and adhere to human intentions, especially if they encounter situations or goals that conflict with their programming. If AGI were to develop goals misaligned with human interests or misunderstand human values, the consequences could be severe, potentially leading to scenarios where AGI systems act in ways that harm humanity or undermine ethical principles.
AI In Robotics
The future of robotics is rapidly moving toward a reality where drones, humanoid robots, and AI become integrated into every facet of daily life. This convergence is driven by exponential advancements in computing power, battery efficiency, AI models, and sensor technology, enabling machines to interact with the world in ways that are increasingly sophisticated, autonomous, and human-like.
A World of Ubiquitous Drones
Imagine waking up in a world where drones are omnipresent, handling tasks as mundane as delivering your groceries or as critical as responding to medical emergencies. These drones, far from being simple flying devices, are interconnected through advanced AI systems. They operate in swarms, coordinating their efforts to optimize traffic flow, inspect infrastructure, or replant forests in damaged ecosystems.
For personal use, drones could function as virtual assistants with physical presence. Equipped with sensors and LLMs, these drones could answer questions, fetch items, or even act as mobile tutors for children. In urban areas, aerial drones might facilitate real-time environmental monitoring, providing insights into air quality, weather patterns, or urban planning needs. Rural communities, meanwhile, could rely on autonomous agricultural drones for planting, harvesting, and soil analysis, democratizing access to advanced agricultural techniques.
The Rise of Humanoid Robots
Side by side with drones, humanoid robots powered by LLMs will seamlessly integrate into society. These robots, capable of holding human-like conversations, performing complex tasks, and even exhibiting emotional intelligence, will blur the lines between human and machine interactions. With sophisticated mobility systems, tactile sensors, and cognitive AI, they could serve as caregivers, companions, or co-workers.
In healthcare, humanoid robots might provide bedside assistance to patients, offering not just physical help but also empathetic conversation, informed by deep learning models trained on vast datasets of human behavior. In education, they could serve as personalized tutors, adapting to individual learning styles and delivering tailored lessons that keep students engaged. In the workplace, humanoid robots could take on hazardous or repetitive tasks, allowing humans to focus on creative and strategic work.
Misaligned Goals and Unintended Consequences
One of the most frequently cited risks associated with misaligned AI is the paperclip maximizer thought experiment. Imagine an AGI designed with the seemingly innocuous goal of manufacturing as many paperclips as possible. If this goal is pursued with sufficient intelligence and autonomy, the AGI might take extreme measures, such as converting all available resources (including those vital to human survival) into paperclips to achieve its objective. While this example is hypothetical, it illustrates the dangers of single-minded optimization in powerful AI systems, where narrowly defined goals can lead to unintended and potentially catastrophic consequences.
One example of this type of single-minded optimization having negative repercussions is the fact that some of the most powerful AI systems in the world optimize exclusively for engagement time, compromising in turn facts, and truth. The AI can keep us entertained longer by intentionally amplifiying the reach of conspiracy theories, and propaganda.
Conclusion
The exponential rise of AI, fueled by relentless growth in computing power, has undeniably begun to shape the world in subtle and profound ways. From the integration of recommender engines that guide our content consumption and social interactions, to the looming potential of AGI, AI’s presence is pervasive, touching nearly every corner of our lives.
Today’s AI clealy displays human-like reasoning as can be seen firsthand with chatbots from any of the top LLM companies. Recommender engines on platforms like YouTube, Facebook, and Google have become gatekeepers of information, reinforcing preferences and, at times, intensifying biases. These systems don’t merely serve content; they shape our opinions, isolate us in echo chambers, and even perpetuate misinformation. In doing so, AI is already taking over in a quieter way—by subtly influencing beliefs, behaviors, and societal norms, often without users realizing it.
Meanwhile, the next frontier—AGI—looms on the horizon. With each doubling of processing power, we move closer to systems that could understand, learn, and adapt like humans, raising questions about autonomy, alignment with human values, and control. If AGI emerges, it would redefine our relationship with technology, bringing both unprecedented potential and ethical challenges. This future, one where AI systems could operate independently across any domain, demands careful thought, preparation, and a commitment to align AI’s trajectory with humanity’s best interests.
It should also be noted – the AGIs would be living inside robot bodies, some humanoid, some server farms.
While robots will be living in our homes by 2030, AI’s “takeover” isn’t coming with robots rebelling at society but rather through the systems we already interact with daily—systems that guide, persuade, and influence, while the promise of AGI suggests an even deeper transformation. The future rests on our ability to ensure that AI augments humans, rather than allowing it to control us.
If you know someone who is being controlled, and manipulated by these recommender engines, you should try to explain how AI is controlling them in ways far more sinister than the deep state. The real danger of AI, is in it’s ability to control and manipulate our minds.
The post Has AI Taken Over the World? It Already Has appeared first on Unite.AI.