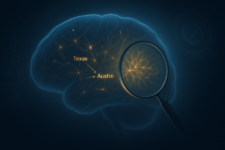
Large language models (LLMs) like Claude have changed the way we use technology. They power tools like chatbots, help write essays and even create poetry. But despite their amazing abilities, these models are still a mystery in many ways. People often call them a “black box” because we can see what they say but not how they figure it out. This lack of understanding creates problems, especially in important areas like medicine or law, where mistakes or hidden biases could cause real harm.
Understanding how LLMs work is essential for building trust. If we can’t explain why a model gave a particular answer, it’s hard to trust its outcomes, especially in sensitive areas. Interpretability also helps identify and fix biases or errors, ensuring the models are safe and ethical. For instance, if a model consistently favors certain viewpoints, knowing why can help developers correct it. This need for clarity is what drives research into making these models more transparent.
Anthropic, the company behind Claude, has been working to open this black box. They’ve made exciting progress in figuring out how LLMs think, and this article explores their breakthroughs in making Claude’s processes easier to understand.
Mapping Claude’s Thoughts
In mid-2024, Anthropic’s team made an exciting breakthrough. They created a basic “map” of how Claude processes information. Using a technique called dictionary learning, they found millions of patterns in Claude’s “brain”—its neural network. Each pattern, or “feature,” connects to a specific idea. For example, some features help Claude spot cities, famous people, or coding mistakes. Others tie to trickier topics, like gender bias or secrecy.
Researchers discovered that these ideas are not isolated within individual neurons. Instead, they’re spread across many neurons of Claude’s network, with each neuron contributing to various ideas. That overlap made Anthropic hard to figure out these ideas in the first place. But by spotting these recurring patterns, Anthropic’s researchers started to decode how Claude organizes its thoughts.
Tracing Claude’s Reasoning
Next, Anthropic wanted to see how Claude uses those thoughts to make decisions. They recently built a tool called attribution graphs, which works like a step-by-step guide to Claude’s thinking process. Each point on the graph is an idea that lights up in Claude’s mind, and the arrows show how one idea flows into the next. This graph lets researchers track how Claude turns a question into an answer.
To better understand the working of attribution graphs, consider this example: when asked, “What’s the capital of the state with Dallas?” Claude has to realize Dallas is in Texas, then recall that Texas’s capital is Austin. The attribution graph showed this exact process—one part of Claude flagged “Texas,” which led to another part picking “Austin.” The team even tested it by tweaking the “Texas” part, and sure enough, it changed the answer. This shows Claude isn’t just guessing—it’s working through the problem, and now we can watch it happen.
Why This Matters: An Analogy from Biological Sciences
To see why this matters, it is convenient to think about some major developments in biological sciences. Just as the invention of the microscope allowed scientists to discover cells – the hidden building blocks of life – these interpretability tools are allowing AI researchers to discover the building blocks of thought inside models. And just as mapping neural circuits in the brain or sequencing the genome paved the way for breakthroughs in medicine, mapping the inner workings of Claude could pave the way for more reliable and controllable machine intelligence. These interpretability tools could play a vital role, helping us to peek into the thinking process of AI models.
The Challenges
Even with all this progress, we’re still far from fully understanding LLMs like Claude. Right now, attribution graphs can only explain about one in four of Claude’s decisions. While the map of its features is impressive, it covers just a portion of what’s going on inside Claude’s brain. With billions of parameters, Claude and other LLMs perform countless calculations for every task. Tracing each one to see how an answer forms is like trying to follow every neuron firing in a human brain during a single thought.
There’s also the challenge of “hallucination.” Sometimes, AI models generate responses that sound plausible but are actually false—like confidently stating an incorrect fact. This occurs because the models rely on patterns from their training data rather than a true understanding of the world. Understanding why they veer into fabrication remains a difficult problem, highlighting gaps in our understanding of their inner workings.
Bias is another significant obstacle. AI models learn from vast datasets scraped from the internet, which inherently carry human biases—stereotypes, prejudices, and other societal flaws. If Claude picks up these biases from its training, it may reflect them in its answers. Unpacking where these biases originate and how they influence the model’s reasoning is a complex challenge that requires both technical solutions and careful consideration of data and ethics.
The Bottom Line
Anthropic’s work in making large language models (LLMs) like Claude more understandable is a significant step forward in AI transparency. By revealing how Claude processes information and makes decisions, they’re forwarding towards addressing key concerns about AI accountability. This progress opens the door for safe integration of LLMs into critical sectors like healthcare and law, where trust and ethics are vital.
As methods for improving interpretability develop, industries that have been cautious about adopting AI can now reconsider. Transparent models like Claude provide a clear path to AI’s future—machines that not only replicate human intelligence but also explain their reasoning.
The post How Does Claude Think? Anthropic’s Quest to Unlock AI’s Black Box appeared first on Unite.AI.