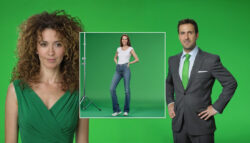
Despite community and investor enthusiasm around visual generative AI, the output from such systems is not always ready for real-world usage; one example is that gen AI systems tend to output entire images (or a series of images, in the case of video), rather than the individual, isolated elements that are typically required for diverse applications in multimedia, and for visual effects practitioners.
A simple example of this is clip-art designed to ‘float’ over whatever target background the user has selected:
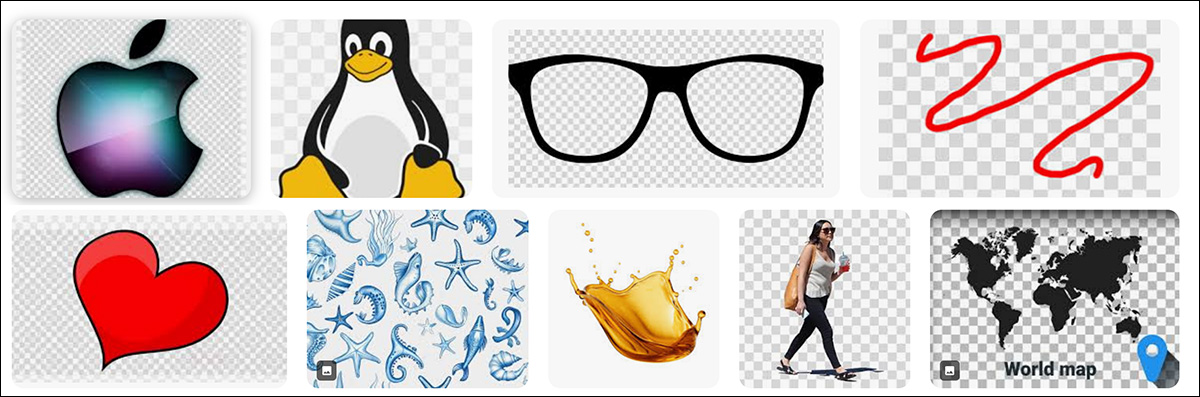
The light-grey checkered background, perhaps most familiar to Photoshop users, has come to represent the alpha channel, or transparency channel, even in simple consumer items such as stock images.
Transparency of this kind has been commonly available for over thirty years; since the digital revolution of the early 1990s, users have been able to extract elements from video and images through an increasingly sophisticated series of toolsets and techniques.
For instance, the challenge of ‘dropping out’ blue-screen and green-screen backgrounds in video footage, once the purview of expensive chemical processes and optical printers (as well as hand-crafted mattes), would become the work of minutes in systems such as Adobe’s After Effects and Photoshop applications (among many other free and proprietary programs and systems).
Once an element has been isolated, an alpha channel (effectively a mask that obscures any non-relevant content) allows any element in the video to be effortlessly superimposed over new backgrounds, or composited together with other isolated elements.
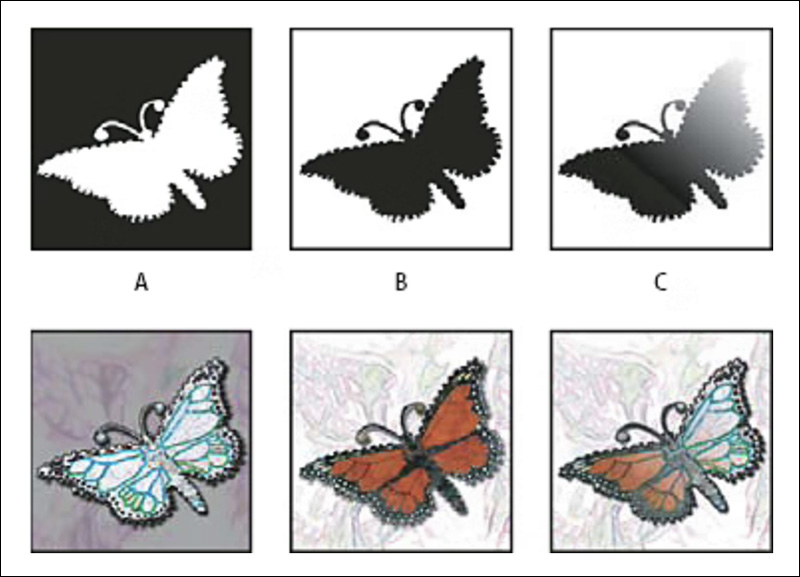
Examples of alpha channels, with their effects depicted in the lower row. Source: https://helpx.adobe.com/photoshop/using/saving-selections-alpha-channel-masks.html
Dropping Out
In computer vision, the creation of alpha channels falls within the aegis of semantic segmentation, with open source projects such as Meta’s Segment Anything providing a text-promptable method of isolating/extracting target objects, through semantically-enhanced object recognition.
The Segment Anything framework has been used in a wide range of visual effects extraction and isolation workflows, such as the Alpha-CLIP project.
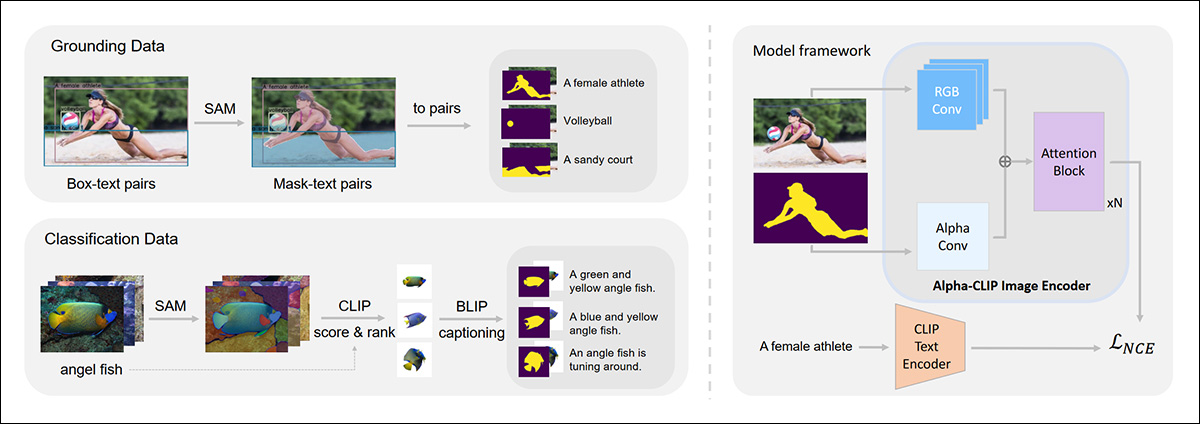
Example extractions using Segment Anything, in the Alpha-CLIP framework: Source: https://arxiv.org/pdf/2312.03818
There are many alternative semantic segmentation methods that can be adapted to the task of assigning alpha channels.
However, semantic segmentation relies on trained data which may not contain all the categories of object that are required to be extracted. Although models trained on very high volumes of data can enable a wider range of objects to be recognized (effectively becoming foundational models, or world models), they are nonetheless limited by the classes that they are trained to recognize most effectively.
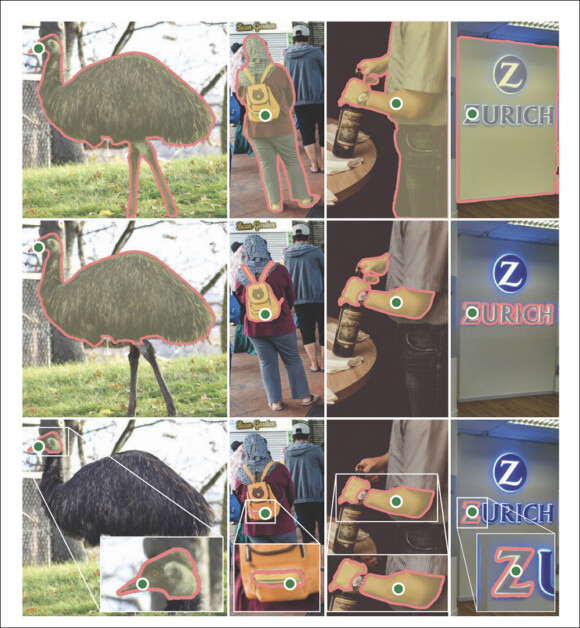
Semantic segmentation systems such as Segment Anything can struggle to identify certain objects, or parts of objects, as exemplified here in output from ambiguous prompts. Source: https://maucher.pages.mi.hdm-stuttgart.de/orbook/deeplearning/SAM.html
In any case, semantic segmentation is just as much a post facto process as a green screen procedure, and must isolate elements without the advantage of a single swathe of background color that can be effectively recognized and removed.
For this reason, it has occasionally occurred to the user community that images and videos could be generated which actually contain green screen backgrounds that could be instantly removed via conventional methods.
Unfortunately, popular latent diffusion models such as Stable Diffusion often have some difficulty rendering a really vivid green screen. This is because the models’ training data does not typically contain a great many examples of this rather specialized scenario. Even when the system succeeds, the idea of ‘green’ tends to spread in an unwanted manner to the foreground subject, due to concept entanglement:
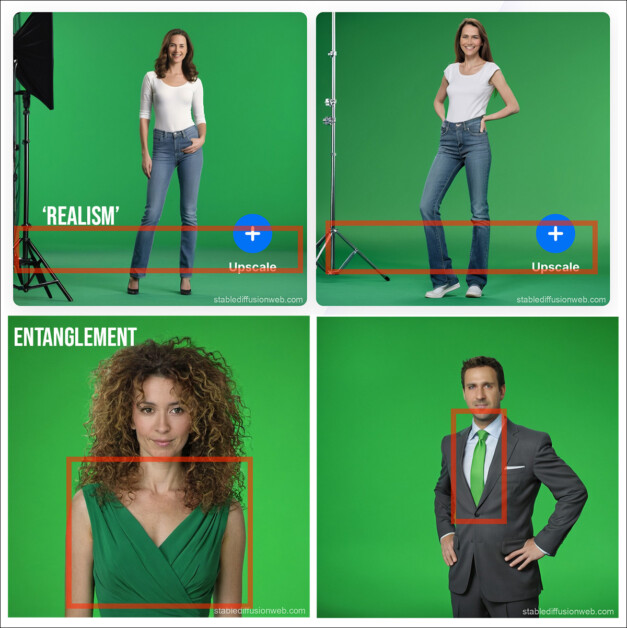
Above, we see that Stable Diffusion has prioritized authenticity of image over the need to create a single intensity of green, effectively replicating real-world problems that occur in traditional green screen scenarios. Below, we see that the ‘green’ concept has polluted the foreground image. The more the prompt focuses on the ‘green’ concept, the worse this problem is likely to get. Source: https://stablediffusionweb.com/
Despite the advanced methods in use, both the woman’s dress and the man’s tie (in the lower images seen above) would tend to ‘drop out’ along with the green background – a problem that hails back* to the days of photochemical emulsion dye removal in the 1970s and 1980s.
As ever, the shortcomings of a model can be overcome by throwing specific data at a problem, and devoting considerable training resources. Systems such as Stanford’s 2024 offering LayerDiffuse create a fine-tuned model capable of generating images with alpha channels:
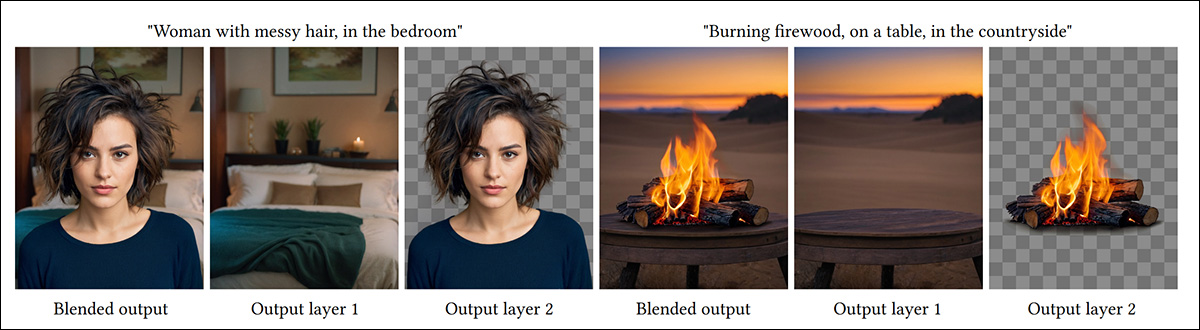
The Stanford LayerDiffuse project was trained on a million apposite images capable of imbuing the model with transparency capabilities. Source: https://arxiv.org/pdf/2402.17113
Unfortunately, in addition to the considerable curation and training resources required for this approach, the dataset used for LayerDiffuse is not publicly available, restricting the usage of models trained on it. Even if this impediment did not exist, this approach is difficult to customize or develop for specific use cases.
A little later in 2024, Adobe Research collaborated with Stonybrook University to produce MAGICK, an AI extraction approach trained on custom-made diffusion images.
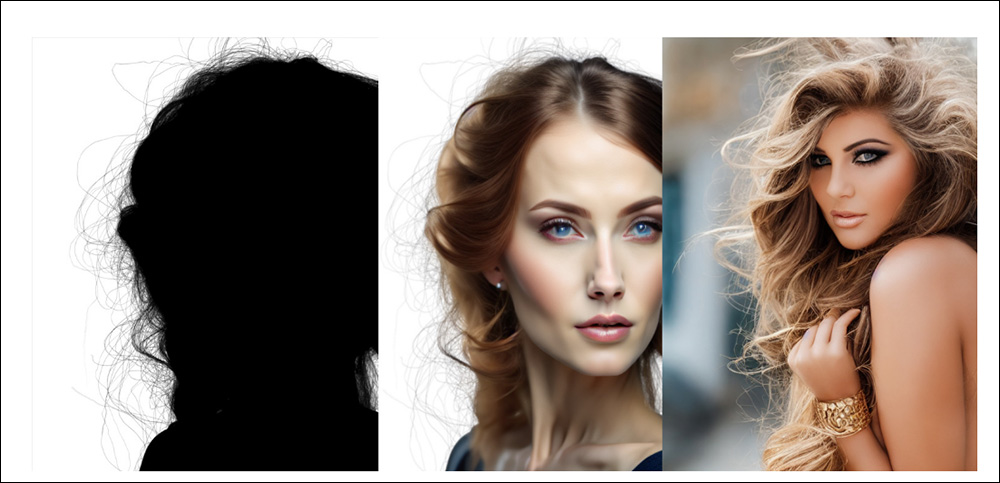
From the 2024 paper, an example of fine-grained alpha channel extraction in MAGICK. Source: https://openaccess.thecvf.com/content/CVPR2024/papers/Burgert_MAGICK_A_Large-scale_Captioned_Dataset_from_Matting_Generated_Images_using_CVPR_2024_paper.pdf
150,000 extracted, AI-generated objects were used to train MAGICK, so that the system would develop an intuitive understanding of extraction:
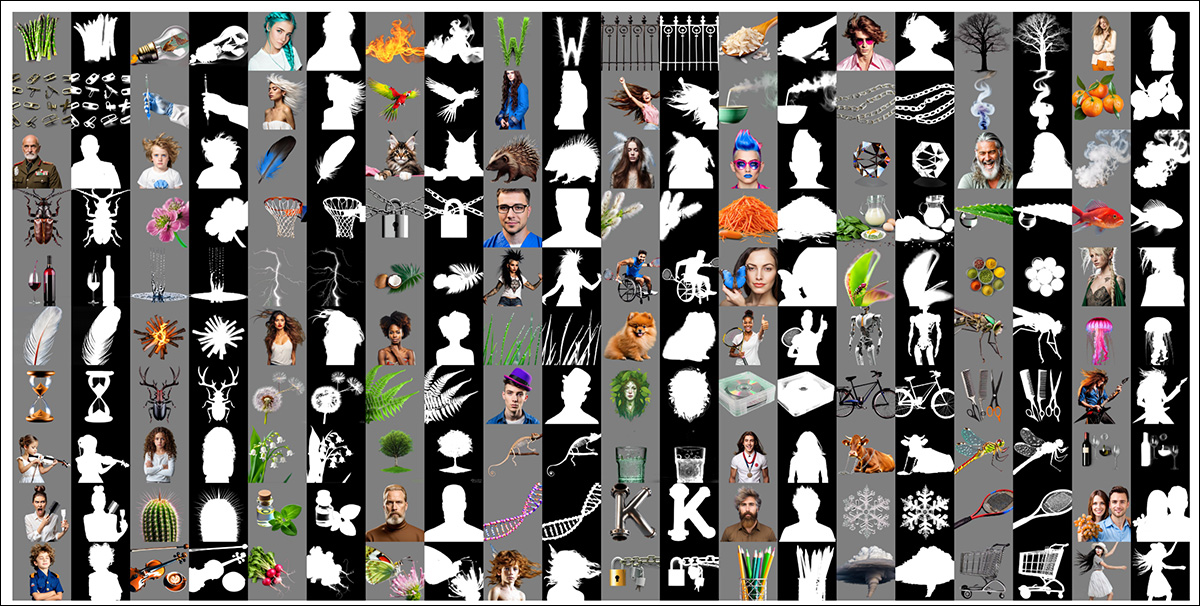
Samples from the MAGICK training dataset.
This dataset, as the source paper states, was very difficult to generate for the aforementioned reason – that diffusion methods have difficulty creating solid keyable swathes of color. Therefore, manual selection of the generated mattes was necessary.
This logistic bottleneck once again leads to a system that cannot be easily developed or customized, but rather must be used within its initially-trained range of capability.
TKG-DM – ‘Native’ Chroma Extraction for a Latent Diffusion Model
A new collaboration between German and Japanese researchers has proposed an alternative to such trained methods, capable – the paper states – of obtaining better results than the above-mentioned methods, without the need to train on specially-curated datasets.

TKG-DM alters the random noise that seeds a generative image so that it is better-capable of producing a solid, keyable background – in any color. Source: https://arxiv.org/pdf/2411.15580
The new method approaches the problem at the generation level, by optimizing the random noise from which an image is generated in a latent diffusion model (LDM) such as Stable Diffusion.
The approach builds on a previous investigation into the color schema of a Stable Diffusion distribution, and is capable of producing background color of any kind, with less (or no) entanglement of the key background color into foreground content, compared to other methods.
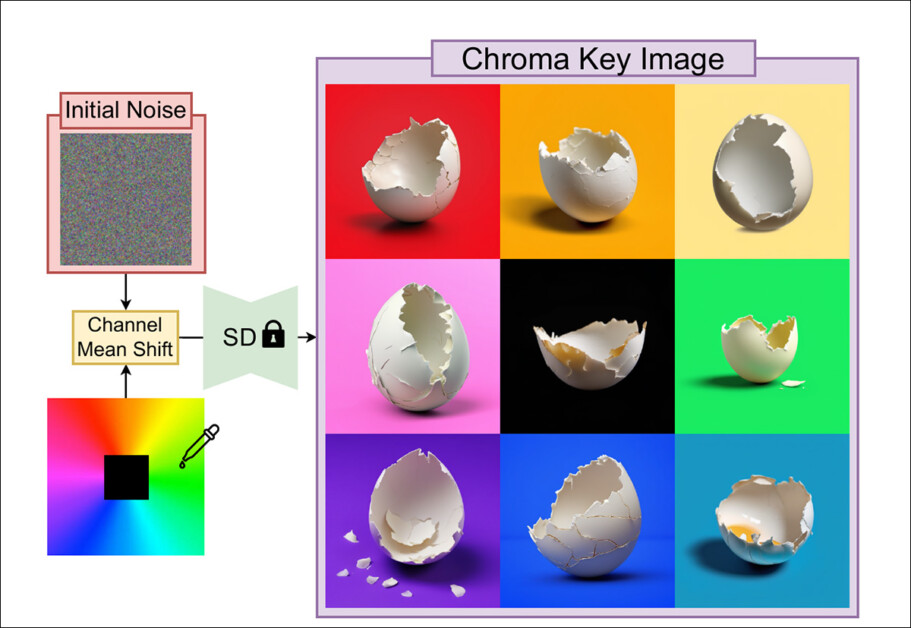
Initial noise is conditioned by a channel mean shift that is able to influence aspects of the denoising process, without entangling the color signal into the foreground content.
The paper states:
‘Our extensive experiments demonstrate that TKG-DM improves FID and mask-FID scores by 33.7% and 35.9%, respectively.
‘Thus, our training-free model rivals fine-tuned models, offering an efficient and versatile solution for various visual content creation tasks that require precise foreground and background control. ‘
The new paper is titled TKG-DM: Training-free Chroma Key Content Generation Diffusion Model, and comes from seven researchers across Hosei University in Tokyo and RPTU Kaiserslautern-Landau & DFKI GmbH, in Kaiserslautern.
Method
The new approach extends the architecture of Stable Diffusion by conditioning the initial Gaussian noise through a channel mean shift (CMS), which produces noise patterns designed to encourage the desired background/foreground separation in the generated result.
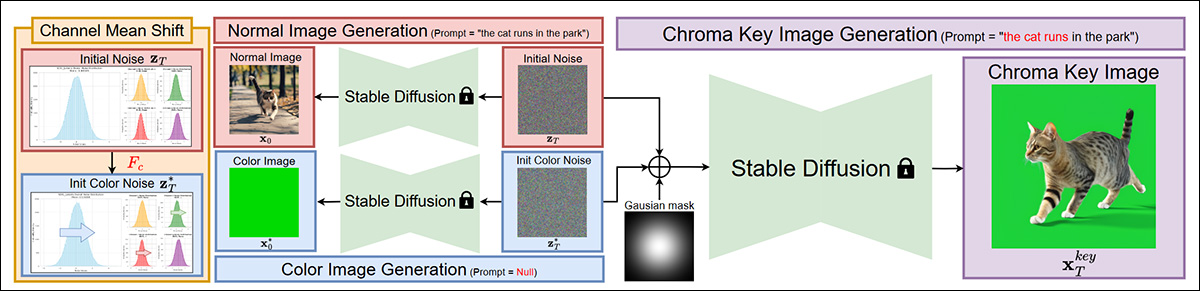
Schema for the the proposed system.
CMS adjusts the mean of each color channel while maintaining the general development of the denoising process.
The authors explain:
‘To generate the foreground object on the chroma key background, we apply an init noise selection strategy that selectively combines the initial [noise] and the init color [noise] using a 2D Gaussian [mask].
‘This mask creates a gradual transition by preserving the original noise in the foreground region and applying the color-shifted noise to the background region.’
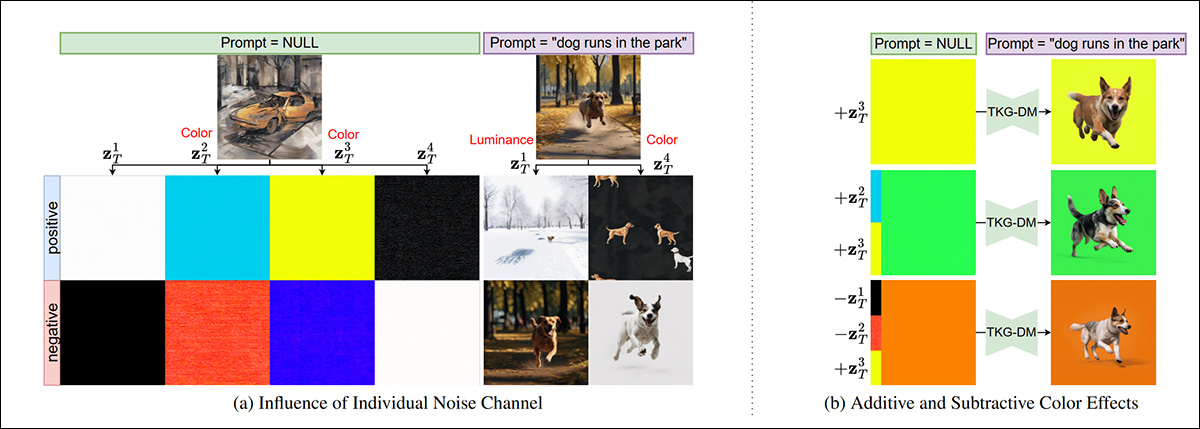
The color channel desired for the background chroma color is instantiated with a null text prompt, while the actual foreground content is created semantically, from the user’s text instruction.
Self-attention and cross-attention are used to separate the two facets of the image (the chroma background and the foreground content). Self-attention helps with internal consistency of the foreground object, while cross-attention maintains fidelity to the text prompt. The paper points out that since background imagery is usually less detailed and emphasized in generations, its weaker influence is relatively easy to overcome and substitute with a swatch of pure color.
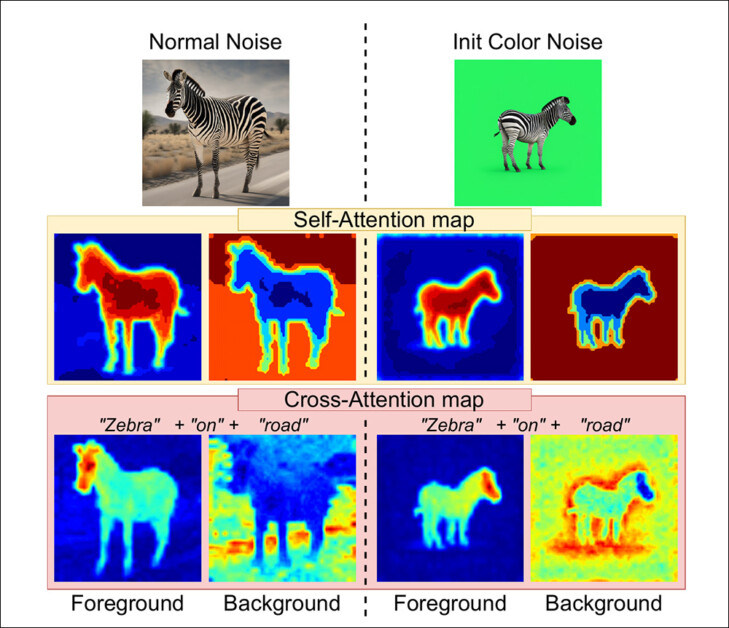
A visualization of the influence of self-attention and cross-attention in the chroma-style generation process.
Data and Tests
TKG-DM was tested using Stable Diffusion V1.5 and Stable Diffusion SDXL. Images were generated at 512x512px and 1024x1024px, respectively.
Images were created using the DDIM scheduler native to Stable Diffusion, at a guidance scale of 7.5, with 50 denoising steps. The targeted background color was green, now the dominant dropout method.
The new approach was compared to DeepFloyd, under the settings used for MAGICK; to the fine-tuned low-rank diffusion model GreenBack LoRA; and also to the aforementioned LayerDiffuse.
For the data, 3000 images from the MAGICK dataset were used.
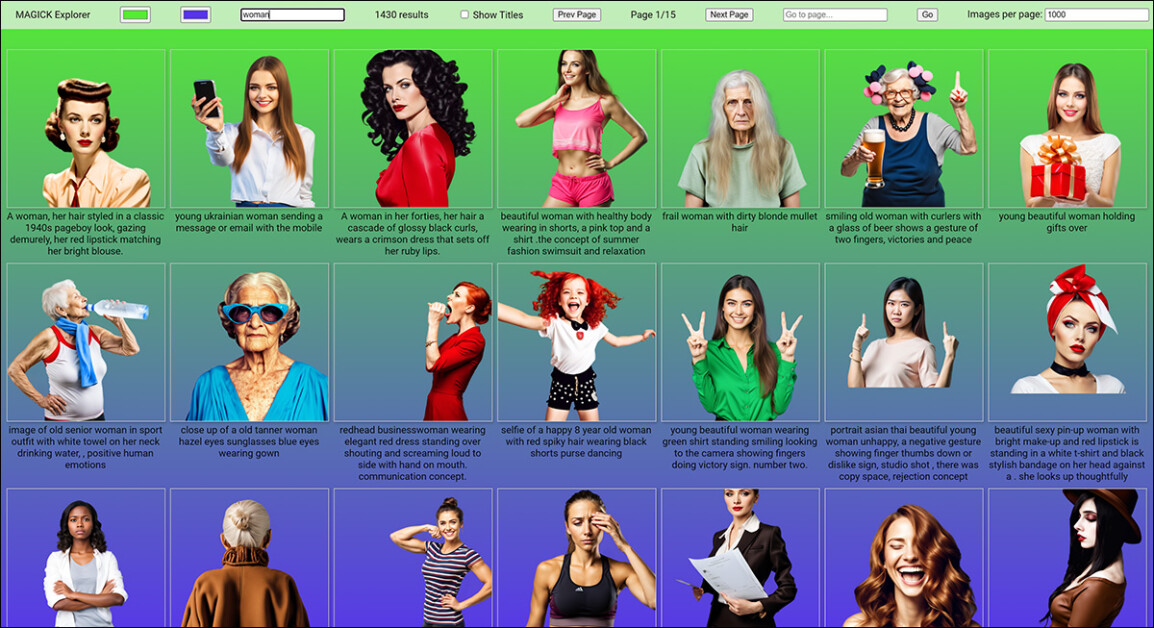
Examples from the MAGICK dataset, from which 3000 images were curated in tests for the new system. Source: https://ryanndagreat.github.io/MAGICK/Explorer/magick_rgba_explorer.html
For metrics, the authors used Fréchet Inception Distance (FID) to assess foreground quality. They also developed a project-specific metric called m-FID, which uses the BiRefNet system to assess the quality of the resulting mask.
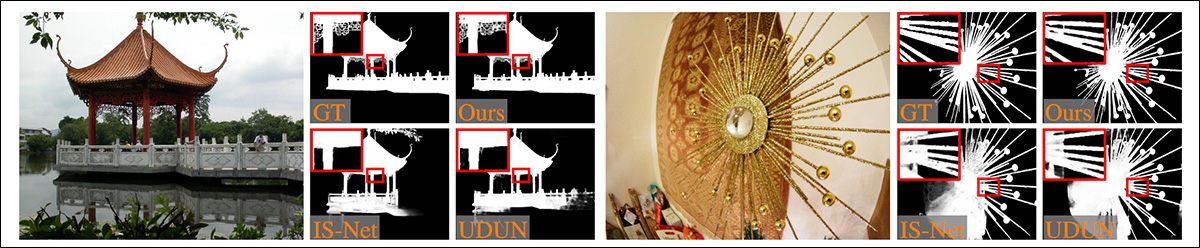
Visual comparisons of the BiRefNet system against prior methods. Source: https://arxiv.org/pdf/2401.03407
To test semantic alignment with the input prompts, the CLIP-Sentence (CLIP-S) and CLIP-Image (CLIP-I) methods were used. CLIP-S evaluates prompt fidelity, and CLIP-I the visual similarity to ground truth.
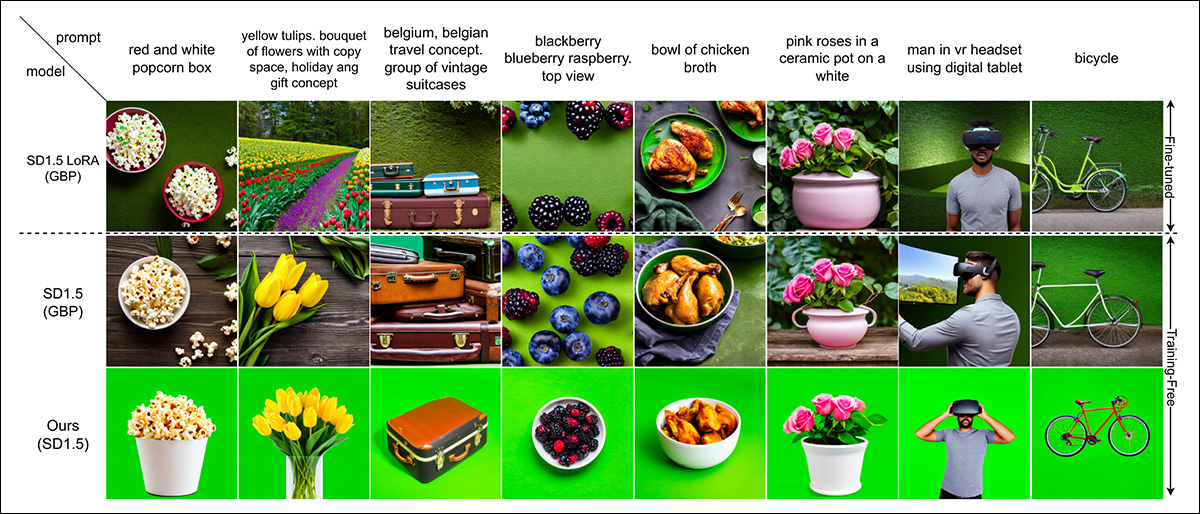
First set of qualitative results for the new method, this time for Stable Diffusion V1.5. Please refer to source PDF for better resolution.
The authors assert that the results (visualized above and below, SD1.5 and SDXL, respectively) demonstrate that TKG-DM obtains superior results without prompt-engineering or the necessity to train or fine-tune a model.
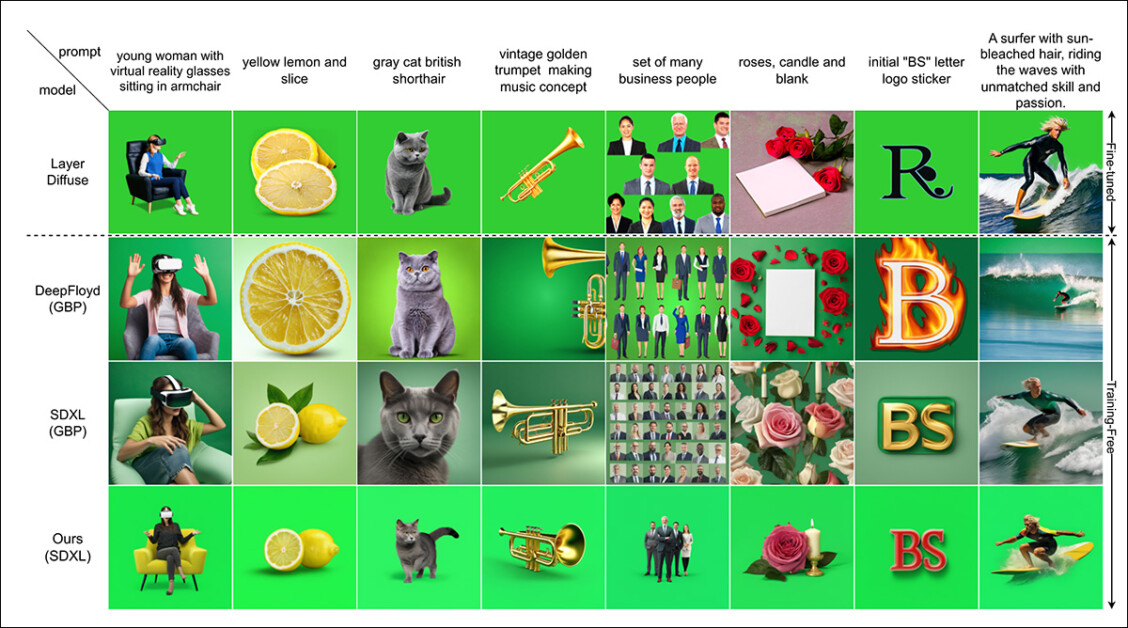
SDXL qualitative results. Please refer to source PDF for better resolution.
They observe that with a prompt to incite a green background in the generated results, Stable Diffusion 1.5 has difficulty generating a clean background, while SDXL (though performing a little better) produces unstable light green tints liable to interfere with separation in a chroma process.
They further note that while LayerDiffuse generates well-separated backgrounds, it occasionally loses detail, such as precise numbers or letters, and the authors attribute this to limitations in the dataset. They add that mask generation also occasionally fails, leading to ‘uncut’ images.
For quantitative tests, though LayerDiffuse apparently has the advantage in SDXL for FID, the authors emphasize that this is the result of a specialized dataset that effectively constitutes a ‘baked’ and non-flexible product. As mentioned earlier, any objects or classes not covered in that dataset, or inadequately covered, may not perform as well, while further fine-tuning to accommodate novel classes presents the user with a curation and training burden.
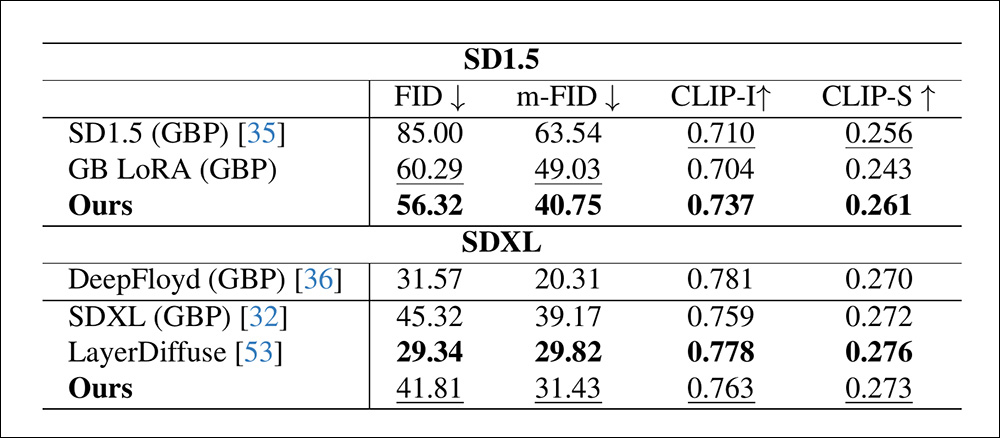
Quantitative results for the comparisons. LayerDiffuse’s apparent advantage, the paper implies, comes at the expense of flexibility, and the burden of data curation and training.
The paper states:
‘DeepFloyd’s high FID, m-FID, and CLIP-I scores reflect its similarity to the ground truth based on DeepFloyd’s outputs. However, this alignment gives it an inherent advantage, making it unsuitable as a fair benchmark for image quality. Its lower CLIP-S score further indicates weaker text alignment compared to other models.
Overall, these results underscore our model’s ability to generate high-quality, text-aligned foregrounds without fine-tuning, offering an efficient chroma key content generation solution.’
Finally, the researchers conducted a user study to evaluate prompt adherence across the various methods. A hundred participants were asked to judge 30 image pairs from each method, with subjects extracted using BiRefNet and manual refinements across all examples. The authors’ training-free approach was preferred in this study.
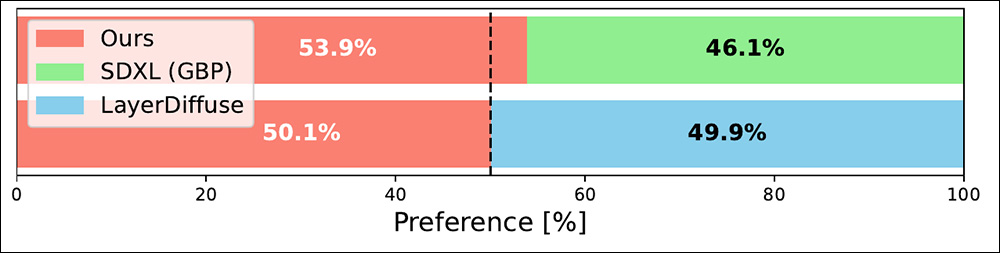
Results from the user study.
TKG-DM is compatible with the popular ControlNet third-party system for Stable Diffusion, and the authors contend that it produces superior results to ControlNet’s native ability to achieve this kind of separation.
Conclusion
Perhaps the most notable takeaway from this new paper is the extent to which latent diffusion models are entangled, in contrast to the popular public perception that they can effortlessly separate facets of images and videos when generating new content.
The study further emphasizes the extent to which the research and hobbyist community has turned to fine-tuning as a post facto fix for models’ shortcomings – a solution which will always address specific classes and types of object. In such a scenario, a fine-tuned model will either work very well on a limited number of classes, or else work tolerably well on a much more higher volume of possible classes and objects, according to higher amounts of data in the training sets.
Therefore it is refreshing to see at least one solution that does not rely on such laborious and arguably disingenuous solutions.
* Shooting the 1978 movie Superman, actor Christopher Reeve was required to wear a turquoise Superman costume for blue-screen process shots, to avoid the iconic blue costume being erased. The costume’s blue color was later restored via color-grading.
The post Improving Green Screen Generation for Stable Diffusion appeared first on Unite.AI.