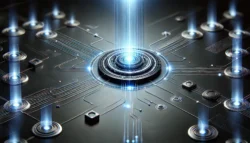
As the currency of the future, collecting data is a familiar process for companies. However, the previous era of technologies and toolsets restricted businesses to simple, structured data, such as transactional information and customer and call center conversations. From there, brands would use sentiment analysis to see how customers felt about a product or service.
New AI tools and capabilities present an incredible opportunity for companies to go beyond structured data and tap into complex and unstructured datasets, unlocking even greater value for customers. For instance, large language models (LLMs) can analyze human interactions and extract crucial insights that enrich customer experience (CX).
Nevertheless, before organizations can harness the power of AI, there are many steps to prepare for an AI integration, and one of the most important (and easily overlooked) is modernizing their data ecosystem. Below are some of the best practices and strategies businesses can leverage to make their data ecosystems AI-ready.
Mastering the Data Estate
Businesses must gather and organize their data into a central repository or data estate to become AI-ready. A company’s data estate is the infrastructure that stores and manages all data, with the primary goal to make data readily available to the right people when they need it to make data-driven decisions or gain a holistic view of their data assets. Unfortunately, most companies do not understand their existing data estate, whether because of legacy constraints, siloed data, poor access control or some combination of reasons.
For businesses to achieve a deeper understanding of their data estate, they should work with a partner that can provide AI solutions, like a unified generative AI orchestration platform. Such a platform can enable enterprises to hasten experimentation and innovation across LLMs, AI-native applications, custom add-ons and — most importantly — data stores. This platform can also function as a secure, scalable and customizable AI workbench, helping companies reach a greater understanding of their data ecosystem, improving AI-driven business solutions.
Having a deeper understanding of one’s data estate not only enhances the effectiveness of AI solutions but also helps organizations use their AI tools more responsibly and in a way that prioritizes data security. Data continues to become more detailed thanks to AI-powered processes and capabilities, underscoring the need for technical conformity with security requirements and adherence to responsible AI best practices.
Elevating Data Governance and Security
Businesses’ data governance frameworks must undergo a significant facelift to be AI-ready. Data governance frameworks are a relatively recent invention focused on more traditional data assets. However, today, in addition to structured data, businesses need to use unstructured data such as personally identifiable information (PII), emails, customer feedback, etc., which current data governance frameworks can’t handle.
Also, generative AI (Gen AI) is changing the data governance paradigm from rule-based to guardrails. Businesses need to define boundaries, rather than relying on hard rules since one success or failure doesn’t reveal anything particularly insightful. By defining boundaries, calculating a probability success rate on a specific set of data and then measuring if outputs remained within those parameters, organizations can determine if an AI solution is technically conforming or if it needs fine tuning.
Organizations must implement and adopt new data governance tools, approaches and methodologies. Leading brands use machine learning techniques to automate data governance and quality assurance. In particular, by establishing policies and thresholds beforehand, these companies can more easily automate the enforcement of data standards. Other best data governance practices include deploying rigorous data processing and storage protocols, anonymizing data where possible and restricting unwarranted data collection.
As the current regulatory landscape around AI-powered data collection continues to evolve, non-compliance could cause serious fines and reputational damage. Navigating these emerging rules will require a comprehensive data governance framework that notes those data protection laws specific to a company’s regions of operation, such as the EU’s AI Act.
Likewise, businesses must improve data literacy across the organization. Companies need to make changes at every level, not just with technical people, like engineers or data scientists. Start with a data maturity assessment, evaluating the data security competencies across different roles. Such an assessment can ferret out if, for example, teams aren’t speaking the same business language. After establishing a baseline, businesses can implement plans to boost data literacy and security awareness.
Enhancing Data Processing Capabilities
If it wasn’t already apparent, unstructured data is the hill brands will fail or succeed on. As mentioned earlier, unstructured data can include PII, emails and customer feedback and any data that can’t get stored in a regular text file, PDF, Microsoft Excel spreadsheet, etc. This unwieldy nature of unstructured data makes it more difficult to analyze or conduct searches. Most data technology tools and platforms cannot incorporate and act upon heavily unstructured data — especially within the context of day-to-day customer interactions.
To overcome unstructured data challenges, organizations must capture this undocumented knowledge, extract it and map it onto an enterprise knowledge base to create a complete picture of their data ecosystem. In the past, this knowledge management process was labor intensive, but AI is making it easier and affordable by collecting data from multiple sources, fixing inconsistencies, removing duplicates, separating important from unimportant data, etc.
Once AI integrates with a data ecosystem, it can help automate the processing of complex assets, such as legal documents, contracts, call center interactions, etc. AI can also help build knowledge graphs to organize unstructured data, making Gen AI capabilities more effective. Moreover, Gen AI enables companies to collect and categorize data based on shared similarities, uncovering missing dependencies.
While these emerging AI-powered data analytics tools can make sense of and draw insights from messy or unorganized data, businesses must also modernize their tech stack to support these complex datasets. Reinvigorating the tech stack starts with an audit — specifically, an assessment of what systems are performing at a level that can jive with modern innovations, and which are not up to par. Companies must also determine which existing systems can integrate with new tools.
Getting Help to Become AI-Ready
Getting a data ecosystem AI-ready is an involved, tedious and multistage process that requires a high level of expertise. Few companies possess such knowledge or skills in-house. If a brand elects to leverage a partner’s expertise to prepare its data ecosystem for AI integration, there are specific qualities they should prioritize in their search.
For starters, an ideal partner must possess technical expertise in multiple, interconnected disciplines (not just AI), such as cloud, security, data, CX, etc. Another telltale sign of a superb partner is if it recognizes the importance of agility. As technological change accelerates, it is getting more challenging to predict the future. To that end, an ideal partner shouldn’t attempt to guess at some future state; rather, it helps a business’ data ecosystem and human capital become agile enough to adapt according to market trends and customer demands.
Additionally, as discussed above, AI technologies apply to everyone, not just the data science team. AI enablement is an organization-wide endeavor. Every employee needs to be AI-literate, regardless of their level. A partner should help bridge this gap, bringing together business and people expertise to help enterprises develop the necessary capabilities in-house.
The post Is Your Data Ecosystem AI-Ready? How Companies Can Ensure Their Systems Are Prepared for an AI Overhaul appeared first on Unite.AI.