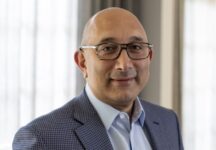
Molham is the Chief Executive Officer of RelationalAI. He has more than 30 years of experience in leading organizations that develop and implement high-value machine learning and artificial intelligence solutions across various industries. Prior to RelationalAI he was CEO of LogicBlox and Predictix (now Infor), CEO of Optimi (now Ericsson), and co-founder of Brickstream (now FLIR). Molham also held senior leadership positions at HNC Software (now FICO) and Retek (now Oracle).
RelationalAI brings together decades of experience in industry, technology, and product development to advance the first and only real cloud-native knowledge graph data management system to power the next generation of intelligent data applications.
As the founder and CEO of RelationalAI, what was the initial vision that drove you to create the company, and how has that vision evolved over the past seven years?
The initial vision was centered around understanding the impact of knowledge and semantics on the successful deployment of AI. Before we got to where we are today with AI, much of the focus was on machine learning (ML), which involved analyzing vast amounts of data to create succinct models that described behaviors, such as fraud detection or consumer shopping patterns. Over time, it became clear that to deploy AI effectively, there was a need to represent knowledge in a way that was both accessible to AI and capable of simplifying complex systems.
This vision has since evolved with deep learning innovations and more recently, language models and generative AI emerging. These advancements have not changed what our company is doing, but have increased the relevance and importance of their approach, particularly in making AI more accessible and practical for enterprise use.
A recent PwC report estimates that AI could contribute up to $15.7 trillion to the global economy by 2030. In your experience, what are the primary factors that will drive this substantial economic impact, and how should businesses prepare to capitalize on these opportunities?
The impact of AI has already been significant and will undoubtedly continue to skyrocket. One of the key factors driving this economic impact is the automation of intellectual labor.
Tasks like reading, summarizing, and analyzing documents – tasks often performed by highly paid professionals – can now be (mostly) automated, making these services much more affordable and accessible.
To capitalize on these opportunities, businesses need to invest in platforms that can support the data and compute requirements of running AI workloads. It’s important that they can scale up and down cost-effectively on a given platform, while also investing in AI literacy among employees so they can understand how to use these models effectively and efficiently.
As AI continues to integrate into various industries, what do you see as the biggest challenges enterprises face in adopting AI effectively? How does data play a role in overcoming these challenges?
One of the biggest challenges I see is ensuring that industry-specific knowledge is accessible to AI. What we are seeing today is that many enterprises have knowledge dispersed across databases, documents, spreadsheets, and code. This knowledge is often opaque to AI models and does not allow organizations to maximize the value that they could be getting.
A significant challenge the industry needs to overcome is managing and unifying this knowledge, sometimes referred to as semantics, to make it accessible to AI systems. By doing this, AI can be more effective in specific industries and within the enterprise as they can then leverage their unique knowledge base.
You’ve mentioned that the future of generative AI adoption will require a combination of techniques such as Retrieval-Augmented Generation (RAG) and agentic architectures. Can you elaborate on why these combined approaches are necessary and what benefits they bring?
It’s going to take different techniques like GraphRAG and agentic architectures to create AI-driven systems that are not only more accurate but also capable of handling complex information retrieval and processing tasks.
Many are finally starting to realize that we are going to need more than one technique as we continue to evolve with AI but rather leveraging a combination of models and tools. One of those is agentic architectures, where you have agents with different capabilities that are helping tackle a complex problem. This technique breaks it up into pieces that you farm out to different agents to achieve the results you want.
There’s also retrieval augmented generation (RAG) that helps us extract information when using language models. When we first started working with RAG, we were able to answer questions whose answers could be found in one part of a document. However, we quickly found out that the language models have difficulty answering harder questions, especially when you have information spread out in various locations in long documents and across documents. So this is where GraphRAG comes into play. By leveraging language models to create knowledge graph representations of information, it can then access the information we need to achieve the results we need and reduce the chances of errors or hallucinations.
Data unification is a critical topic in driving AI value within organizations. Can you explain why unified data is so important for AI, and how it can transform decision-making processes?
Unified data ensures that all the knowledge an enterprise has – whether it’s in documents, spreadsheets, code, or databases – is accessible to AI systems. This unification means that AI can effectively leverage the specific knowledge unique to an industry, sub-industry, or even a single enterprise, making the AI more relevant and accurate in its outputs.
Without data unification, AI systems can only operate on fragmented pieces of knowledge, leading to incomplete or inaccurate insights. By unifying data, we make sure that AI has a complete and coherent picture, which is pivotal for transforming decision-making processes and driving real value within organizations.
How does RelationalAI’s approach to data, particularly with its relational knowledge graph system, help enterprises achieve better decision-making outcomes?
RelationalAI’s data-centric architecture, particularly our relational knowledge graph system, directly integrates knowledge with data, making it both declarative and relational. This approach contrasts with traditional architectures where knowledge is embedded in code, complicating access and understanding for non-technical users.
In today’s competitive business environment, fast and informed decision-making is imperative. However, many organizations struggle because their data lacks the necessary context. Our relational knowledge graph system unifies data and knowledge, providing a comprehensive view that allows humans and AI to make more accurate decisions.
For example, consider a financial services firm managing investment portfolios. The firm needs to analyze market trends, client risk profiles, regulatory changes, and economic indicators. Our knowledge graph system can rapidly synthesize these complex, interrelated factors, enabling the firm to make timely and well-informed investment decisions that maximize returns while managing risk.
This approach also reduces complexity, enhances portability, and minimizes dependence on specific technology vendors, providing long-term strategic flexibility in decision-making.
The role of the Chief Data Officer (CDO) is growing in importance. How do you see the responsibilities of CDOs evolving with the rise of AI, and what key skills will be essential for them moving forward?
The role of the CDO is rapidly evolving, especially with the rise of AI. Traditionally, the responsibilities that now fall under the CDO were managed by the CIO or CTO, focusing primarily on technology operations or the technology produced by the company. However, as data has become one of the most valuable assets for modern enterprises, the CDO’s role has become distinct and crucial.
The CDO is responsible for ensuring the privacy, accessibility, and monetization of data across the organization. As AI continues to integrate into business operations, the CDO will play a pivotal role in managing the data that fuels AI models, ensuring that this data is clean, accessible, and used ethically.
Key skills for CDOs moving forward will include a deep understanding of data governance, AI technologies, and business strategy. They will need to work closely with other departments, empowering teams that traditionally may not have had direct access to data, such as finance, marketing, and HR, to leverage data-driven insights. This ability to democratize data across the organization will be critical for driving innovation and maintaining a competitive edge.
What role does RelationalAI play in supporting CDOs and their teams in managing the increasing complexity of data and AI integration within organizations?
RelationalAI plays a fundamental role in supporting CDOs by providing the tools and frameworks necessary to manage the complexity of data and AI integration effectively. With the rise of AI, CDOs are tasked with ensuring that data is not only accessible and secure but also that it is leveraged to its fullest potential across the organization.
We help CDOs by offering a data-centric approach that brings knowledge directly to the data, making it accessible and understandable to non-technical stakeholders. This is particularly important as CDOs work to put data into the hands of those in the organization who might not traditionally have had access, such as marketing, finance, and even administrative teams. By unifying data and simplifying its management, RelationalAI enables CDOs to empower their teams, drive innovation, and ensure that their organizations can fully capitalize on the opportunities presented by AI.
RelationalAI emphasizes a data-centric foundation for building intelligent applications. Can you provide examples of how this approach has led to significant efficiencies and savings for your clients?
Our data-centric approach contrasts with the traditional application-centric model, where business logic is often embedded in code, making it difficult to manage and scale. By centralizing knowledge within the data itself and making it declarative and relational, we’ve helped clients significantly reduce the complexity of their systems, leading to greater efficiencies, fewer errors, and ultimately, substantial cost savings.
For instance, Blue Yonder leveraged our technology as a Knowledge Graph Coprocessor inside of Snowflake, which provided the semantic understanding and reasoning capabilities needed to predict disruptions and proactively drive mitigation actions. This approach allowed them to reduce their legacy code by over 80% while offering a scalable and extensible solution.
Similarly, EY Financial Services experienced a dramatic improvement by slashing their legacy code by 90% and reducing processing times from over a month to just several hours. These outcomes highlight how our approach enables businesses to be more agile and responsive to changing market conditions, all while avoiding the pitfalls of being locked into specific technologies or vendors.
Given your experience leading AI-driven companies, what do you believe are the most critical factors for successfully implementing AI at scale in an organization?
From my experience, the most significant factors for successfully implementing AI at scale are ensuring you have a strong foundation of data and knowledge and that your employees, particularly those who are more experienced, take the time to learn and become comfortable with AI tools.
It’s also important not to fall into the trap of extreme emotional reactions – either excessive hype or deep cynicism – around new AI technologies. Instead, I recommend a steady, consistent approach to adopting and integrating AI, focusing on incremental improvements rather than expecting a silver bullet solution.
Thank you for the great interview, readers who wish to learn more should visit RelationalAI.
The post Molham Aref, CEO & Founder of RelationalAI appeared first on Unite.AI.