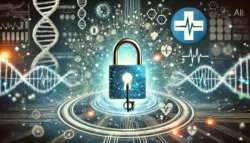
Healthcare in the United States is in the early stages of a significant potential disruption due to the use of Machine Learning and Artificial Intelligence. This shift has been underway for over a decade, but with recent advances, seems poised for more rapid changes. Much work remains to be done to understand the safest and most effective applications of AI in healthcare, to build trust among clinicians in the use of AI, and to adjust our clinical education system to drive better use of AI-based systems.
Applications of AI in Healthcare
AI has been in evolution for decades in healthcare, both in patient-facing and back-office functions. Some of the earliest and most extensive work has occurred in the use of deep learning and computer vision models.
First, some terminology. Traditional statistical approaches in research–e.g. observational studies and clinical trials–have used population-focused modeling approaches that rely on regression models, in which independent variables are used to predict outcomes. In these approaches, while more data is better, there is a plateau effect in which above a certain data set size, no better inferences can be obtained from the data.
Artificial intelligence brings a newer approach to prediction. A structure called a perceptron processes data that is passed forward a row at a time, and is created as a network of layers of differential equations to modify the input data, to produce an output. During training, each row of data as it passes through the network–called a neural network–modifies the equations at each layer of the network so that the predicted output matches the actual output. As the data in a training set is processed, the neural network learns how to predict the outcome.
Several types of networks exist. Convolutional neural networks, or CNNs, were among the first models to find success in healthcare applications. CNNs are very good at learning from images in a process called computer vision and have found applications where image data is prominent: radiology, retinal exams, and skin images.
A newer neural network type called the transformer architecture has become a dominant approach due to its incredible success for text, and combinations of text and images (also called multimodal data). Transformer neural networks are exceptional when given a set of text, at predicting subsequent text. One application of the transformer architecture is the Large Language Model or LLM. Multiple commercial examples of LLMs include Chat GPT, Anthropics Claude, and Metas Llama 3.
What has been observed with neural networks, in general, is that a plateau for improvement in learning has been hard to find. In other words, given more and more data, neural networks continue to learn and improve. The main limits on their capability are larger and larger data sets and the computing power to train the models. In healthcare, the creation of privacy-protecting data sets that faithfully represent true clinical care is a key priority to advance model development.
LLMs may represent a paradigm shift in the application of AI for healthcare. Because of their facility with language and text, they are a good match to electronic records in which almost all data are text. They also do not require highly annotated data for training but can use existing data sets. The two main flaws with these models are that 1) they do not have a world model or an understanding of the data that is being analyzed (they have been called fancy autocomplete), and 2) they can hallucinate or confabulate, making up text or images that appear accurate but create information presented as fact.
Use cases being explored for AI include automation and augmentation for reading of radiology images, retinal images, and other image data; reducing the effort and improving the accuracy of clinical documentation, a major source of clinician burnout; better, more empathic, patient communication; and improving the efficiency of back-office functions like revenue cycle, operations, and billing.
Real-world Examples
AI has been incrementally introduced into clinical care overall. Typically, successful use of AI has followed peer-reviewed trials of performance that have demonstrated success and, in some cases, FDA approval for use.
Among the earliest use cases in which AI performs well have been AI detecting disease in retinal exam images and radiology. For retinal exams, published literature on the performance of these models has been followed by the deployment of automated fundoscopy to detect retinal disease in ambulatory settings. Studies of image segmentation, with many published successes, have resulted in multiple software solutions that provide decision support for radiologists, reducing errors and detecting abnormalities to make radiologist workflows more efficient.
Newer large language models are being explored for assistance with clinical workflows. Ambient voice is being used to enhance the usage of Electronic Health Records (EHRs). Currently, AI scribes are being implemented to aid in medical documentation. This allows physicians to focus on patients while AI takes care of the documentation process, improving efficiency and accuracy.
In addition, hospitals and health systems can use AI’s predictive modeling capabilities to risk-stratify patients, identifying patients who are at high or increasing risk and determining the best course of action. In fact, AI’s cluster detection capabilities are being increasingly used in research and clinical care to identify patients with similar characteristics and determine the typical course of clinical action for them. This can also enable virtual or simulated clinical trials to determine the most effective treatment courses and measure their efficacy.
A future use case may be the use of AI-powered language models in doctor-patient communication. These models have been found to have valid responses for patients that simulate empathetic conversations, making it easier to manage difficult interactions. This application of AI can greatly improve patient care by providing quicker and more efficient triage of patient messages based on the severity of their condition and message.
Challenges and Ethical Considerations
One challenge with AI implementation in healthcare is ensuring regulatory compliance, patient safety, and clinical efficacy when using AI tools. While clinical trials are the standard for new treatments, there is a debate on whether AI tools should follow the same approach. Another concern is the risk of data breaches and compromised patient privacy. Large language models trained on protected data can potentially leak source data, which poses a significant threat to patient privacy. Healthcare organizations must find ways to protect patient data and prevent breaches to maintain trust and confidentiality. Bias in training data is also a critical challenge that needs to be addressed. To avoid biased models, better methods to avoid bias in training data must be introduced. It is crucial to develop training and academic approaches that enable better model training and incorporate equity in all aspects of healthcare to avoid bias.
The use of AI has opened a number of new concerns and frontiers for innovation. Further study of where true clinical benefit may be found in AI use is needed. To address these challenges and ethical concerns, healthcare provider organizations and software companies must focus on developing data sets that accurately model healthcare data while ensuring anonymity and protecting privacy. Additionally, partnerships between healthcare providers, systems, and technology/software companies must be established to bring AI tools into practice in a safe and thoughtful manner. By addressing these challenges, healthcare organizations can harness the potential of AI while upholding patient safety, privacy, and fairness.
The post Unlocking New Possibilities in Healthcare with AI appeared first on Unite.AI.