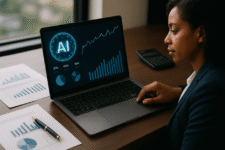
Artificial intelligence (AI) transforms companies’ operations, offering unprecedented opportunities to uncover actionable insights that drive efficiency and measurable results. Companies like GE Aerospace already use AI to analyze complex datasets, improving decision-making and operational performance. By leveraging AI, organizations can analyze vast amounts of data, identify patterns, and make informed decisions more quickly and accurately. AI also enhances decision-making by enabling predictive analytics, automating data analysis, personalizing customer insights, detecting fraud, and optimizing operations. In business intelligence, AI automates data cleanup, detects anomalies, and generates predictive insights that support strategic growth.
The data quality challenge to business intelligence
Business intelligence starts with one core requirement: clean, high-quality data. Without it, even insights generated through AI tools can be misleading or missed entirely. As the volume of data and data sources grows, so do the inconsistencies in formats, inaccuracies, and non-standardized information. Data scientists spend considerable time cleaning the raw data, especially from large repositories like data lakes, making data analysis costly, error-prone, and time-consuming.
For these reasons, AI’s first role in business analysis is to improve and automate data preparation. With its ability to process structured and unstructured data, from images to complex streaming data, AI tools speed up anomaly detection, improve data classification, and standardize formats across data sources. By automating these early-stage tasks, AI reduces the cost and time required for data preparation, freeing analysts to focus on strategy and interpretation, where the actual value of business intelligence lies.
Personalizing customer insights
According to The State of Personalization Report 2024, 89 percent of respondents say, “personalization is crucial to their business’ success in the next three years.” The power of AI technologies like predictive analytics and machine learning-based recommendations enables companies like Spotify and Ikea to tailor recommendations and experiences based on a consumer’s past behaviors. Yet, consumers also have privacy concerns. Another AI approach to personalization is to aggregate and anonymize group behavior data to identify trends and generate recommendations for individuals. This cohort approach provides personalization without compromising privacy.
Some organizations use AI-generated synthetic data to help protect consumer privacy as another option. Synthetic data is realistic data that mimics patterns found in actual datasets without exposing personal details. This method does more than protect privacy—it can address bias where real-world training data might overrepresent certain groups. Generating synthetic data is also helpful in scaling datasets a company wants to use to conduct market analysis, such as analyzing future trends or testing product or pricing changes when its dataset is too small.
Practical AI tools for better business insights
AI can raise business insights to new levels, regardless of the industry. Key technologies include:
- Natural language processing (NLP). One application of NLP enables companies to analyze customer feedback by processing text data to perform sentiment analysis. Analyzing human communication helps companies understand their customers’ state of mind, which they can use to guide product development and service improvements.
- Machine learning for predictive analytics. Machine learning models can forecast sales trends, predict customer churn, and identify potential data gaps, allowing for proactive decision-making. For example, Sparex implemented AI solutions that resulted in a 95 percent improvement in inventory accuracy, a 30 percent reduction in processing time, and annual savings of $5 million.
- AI-generated data visualization. AI platforms like Manus and ai can automatically analyze and create comprehensive data dashboards, reducing the time and effort required for manual dashboard creation. These tools provide instant insights from complex datasets, enabling quicker and more informed business decisions.
As these technologies become more user-friendly and scalable, businesses of all sizes can apply them to gain strategic insights about their operations and markets.
Strategic implementations
Strategic AI implementation begins with a clear-eyed assessment of available data. It’s essential for organizations to define specific business goals, identify relevant data points, and evaluate the quality and accessibility of their existing datasets. From there, align AI tools and platform choices to the business goals.
For example, customer service chatbots are a common entry point. They use NLP to handle routine inquiries and analyze customer feedback to reveal persistent issues. Retailers can use image recognition to monitor product inventory on shelves or analyze how customers interact with displays. For sales or operations teams, predictive analytics tools help forecast demand using historical data, enabling better inventory and resource planning.
Incorporating AI tools for data analytics and insights can be less daunting than organizations might think. No-code platforms offer a fast, low-risk way to get started—ideal for teams without in-house data science and AI expertise. These platforms also let teams test and refine their AI approach before adopting more customized development. It’s vital for companies to weigh their internal resources and the urgency of adoption when considering whether to build their own AI platform. A proprietary in-house tool offers more control, but third-party platforms are faster to deploy. In either case, a phased approach allows organizations to grow internal AI skills and quantify the return on investment in AI before scaling up.
Future trends in AI for business intelligence
As AI tools mature, several emerging trends are poised to expand their business value. For example, synthetic data is growing rapidly, driven by its ability to create diverse, privacy-preserving datasets for training AI models—especially where access to real-world data is limited or sensitive. Another developing area is explainable AI (XAI), which increases transparency by allowing models to articulate how they reach decisions. Finally, advanced computing and analytical methods like Quantum AI and Graph AI are beginning to influence business intelligence. While still early-stage, these approaches promise a more rigorous analysis of complex data relationships and offer users the ability to extract insights through simpler queries. These trends reflect a shift toward AI that is more robust and accessible, ethical, and aligned with evolving business and regulatory expectations.
Human intelligence plus AI
The true power of AI in business intelligence is the collaboration between technology and human insight. By automating data cleaning and processing, AI lets data scientists and analysts focus on strategic thinking and complex problem-solving rather than mundane tasks. Human oversight is essential to provide context, ethical governance, and nuanced interpretation that validate AI-generated insights and correct potential biases. The future of business intelligence combines AI’s computational power with human creativity and critical thinking. Successful organizations will enhance their business insights and decision-making by using AI to amplify human potential rather than replace expertise.
The post Utilizing AI for Better Business Insights: Minimize Costs, Maximize Results appeared first on Unite.AI.